Team
Joshua Vogelstein, Ph.D.
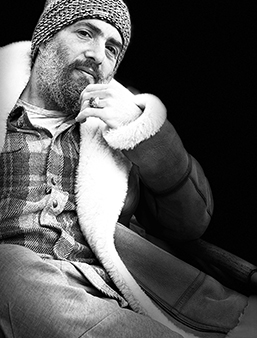
Department of Biomedical Engineering
Johns Hopkins University
jovo@jhu.edu
jovo.me
Download CV
I am an Associate Professor in the Department of Biomedical Engineering at Johns Hopkins University, with joint appointments in Applied Mathematics and Statistics, Computer Science, Electrical and Computer Engineering, Neuroscience, and Biostatistics. Our research focuses primarily on the intersection of natural and artificial intelligence. We develop and apply high-dimensional nonlinear machine learning methods to biomedical big data science challenges. We have published about 200 papers in prominent scientific and engineering venues, with >12,000 citations and an h-index >45. Our group is one of the few in the world that regularly publishes in both top scientific (e.g., Nature, Science, Cell, PNAS, eLife) and top artificial intelligence (e.g., JMLR, Neurips, ICML) venues. We have received funding from the Transformative Research Award from NIH, the NSF CAREER award, Microsoft Research, and many other government, for-profit and nonprofit organizations. I have advised over 60 trainees, and taught about 200 students in my eight years as faculty. In addition to my academic work, I co-founded Global Domain Partners, a quantitative hedge fund that was acquired by Mosaic Investment Partners in 2012, and software startup Gigantum, which was acquired by nVidia in early 2022. I live in the Chesapeake Bay Watershed with my beloved eternal wife and our three children.
- jovo: google scholar, pubmed, arXiv, bioRxiv, github
- open connectome project: website, github
- other links: wiki, twitter, medium, pubpeer, facebook, linkedin,
CV
Personal Information
Primary Appointment
- 02/22 – Associate Professor Department of Biomedical Engineering, JHU.
- 08/14 – 02/22 Assistant Professor Department of Biomedical Engineering, JHU.
Joint Appointments
- 09/19 – Joint Appointment Department of Biostatistics, JHU, Baltimore, MD, USA.
- 08/15 – Joint Appointment Department of Applied Mathematics and Statistics, JHU.
- 08/14 – Joint Appointment Department of Neuroscience, JHU.
- 08/14 – Joint Appointment Department of Computer Science, JHU.
Institutional and Center Appointments
- 08/15 – Steering Committee Kavli Neuroscience Discovery Institute (KNDI).
- 08/14 – Core Faculty Institute for Computational Medicine, JHU.
- 08/14 – Core Faculty Center for Imaging Science, JHU.
- 08/14 – Assistant Research Faculty Human Language Technology Center of Excellence, JHU.
- 10/12 – Affiliated Faculty Institute for Data Intensive Engineering and Sciences, JHU.
Education
- 2003 – 2009 Ph.D in Neuroscience Johns Hopkins School of Medicine
Advisor: Eric Young
Thesis: OOPSI: a family of optical spike inference algorithms for inferring neural connectivity from population calcium imaging
- 2009 – 2009 M.S. in Applied Mathematics & Statistics Johns Hopkins University
- 1998 – 2002 B.A. in Biomedical Engineering Washington University, St. Louis
Training
- 2022 Foundations in Somatic Abolitionism for White Bodies (14 Hours)
- 2022 Sacred Sons Leadership Training Level 1
- 2022 Creatorhood Initiate Training - Phase 1
- 2021 JHU SafeZone training
Academic Experience
- 08/18 – Director of Biomedical Data Science Focus Area Department of Biomedical Engineering, Johns Hopkins University, Baltimore, MD, USA.
- 05/16 – Visiting Scientist Howard Hughes Medical Institute, Janelia Research Campus, Ashburn, VA, USA
- 10/12 – 08/14 Endeavor Scientist Child Mind Institute, New York, NY, USA
- 08/12 – 08/14 Affiliated Faculty Kenan Institute for Ethics, Duke University, Durham, NC, USA
- 08/12 –08/14 Adjunct Faculty Department of Computer Science, JHU, Baltimore, MD, USA
- 12/09 – 01/11 Post-Doctoral Fellow Department of Applied Mathematics and Statistics, Supervised by Carey E.Priebe, JHU, Baltimore, MD, USA
Research Statistics of populations of networks
- 06/01 – 09/01 Research Assistant Prof. Randy O'Reilly, Dept. of Psychology, University of Colorado, Denver, CO, USA
- 06/00 – 09/00 Clinical Engineer Johns Hopkins Hospital, JHU, Baltimore, MD, USA
- 06/99 – 08/99 Research Assistant under Dr. Jeffrey Williams Dept. of Neurosurgery, Johns Hopkins Hospital, Baltimore, MD, USA
- 06/98 – 08/98 Research Assistant under Professor Kathy Cho Dept. of Pathology, Johns Hopkins School of Medicine, Baltimore, MD, USA
Published Peer-Reviewed Research Articles
Note: CV author in bold; Trainees are underlined,
(112 papers; top 10 cited 3,922 times; H-index 36; 12 first, 13 last, 48 middle authorships) as of 2023/10/12
- [112]
S. Panda, S. Cencheng, R. Perry, J. Zorn, A. Lutz, C. E. Priebe, and J. T. Vogelstein. "Universally consistent K-sample tests via dependence measures" Statistics and probability Letters, 2025. [URL]
- [111]
E. W. Bridgeford, M. Powell, G. Kiar, S. Noble, J. Chung, S. Panda, R. Lawrence, T. Xu, M. Milham, B. Caffo, and J. T. Vogelstein. "When no answer is better than a wrong answer: a causal perspective on batch effects" Imaging Neuroscience, 2024. [URL]
- [110]
T. Xu, G. Kiar, X. Zuo, J. T. Vogelstein, and M. P. Milham. "Challenges in Measuring Individual Differences of Brain Function" Imaging Neuroscience, 2024. [URL]
- [109]
Guodong Chen, Jesús Arroyo, Avanti Athreya, Joshua Cape, Joshua T. Vogelstein, Youngser Park, Chris White, Jonathan Larson, Weiwei Yang, and Carey E. Priebe. "Multiple Network Embedding for Anomaly Detection in Time Series of Graphs" Computational Statistics and Data Analysis, 2024. [URL]
- [108]
S. Cencheng, S. Wang, A. Badea, C. E. Priebe, and J. T. Vogelstein. "Discovering the signal subgraph: An iterative screening approach on graphs" Pattern Recognition Letters, 2024. [URL]
- [107]
S. Cencheng, C. Jaewon, M. Ronak, X. Ting, and J. T. Vogelstein. "Independence Testing for Temporal Data" Transactions on Machine Learning Research, 2024. [URL]
- [106]
X. Li, N. Bianchini Esper, L. Ai, S. Giavasis, H. Jin, E. Feczko, T. Xu, J. Clucas, A. Franco, A. Sólon Heinsfeld, A. Adebimpe, J. T. Vogelstein, C. Yan, O. Esteban, R. A. Poldrack, C. Craddock, D. Fair, T. Satterthwaite, G. Kiar, and M. P. Milham. "Moving Beyond Processing and Analysis-Related Variation in Neuroscience" Nature Human Behaviour, 2024. [DOI]
- [105]
H. S. Helm, A. De Silva, J. T. and Vogelstein, C. E. Priebe, and W. Yang. " Approximately Optimal Domain Adaptation with Fisher’s Linear Discriminant" Mathmatics, 2024. [URL]
- [104]
M. Madhyastha, T. Budavari, V. Braverman, J. Vogelstein, and R. Burns. "T-Rex (Tree-Rectangles): Reformulating Decision Tree Traversal as Hyperrectangle Enclosure" 2024 IEEE 40th International Conference on Data Engineering (ICDE), 2024. [URL]
- [103]
Z. Wang, E. Bridgeford, S. Wang, J. T. Vogelstein, and . B. Caffo. "Statistical Analysis of Data Repeatability Measures" International Statistical Review, 2024. [URL]
- [102]
T. Zuzul, E. C. Pahnke, J. Larson, P. Bourke, N. Caurvina, N. P. Shah, F. Amini, J. Weston, Y. Park, J. Vogelstein, C. White, and C. E. Priebe. "Dynamic Silos: Increased Modularity and Decreased Stability in Intraorganizational Communication Networks During the COVID-19 Pandemic" Management Science, 2024. [URL]
- [101]
R. Xiong, A. Koenecke, M. Powell, Z. Shen, J. T. Vogelstein, and S. Athey. "Federated Causal Inference in Heterogeneous Observational Data" Statistics in Medicine, 2023. [DOI]
- [100]
Benjamin D Pedigo, Mike Powell, Eric W Bridgeford, Michael Winding, Carey E Priebe, and Joshua T Vogelstein. "Generative network modeling reveals quantitative definitions of bilateral symmetry exhibited by a whole insect brain connectome" eLife Sciences Publications, Ltd, 2023. [URL]
- [99]
Michael Winding, Benjamin D Pedigo, Christopher L Barnes, Heather G Patsolic, Youngser Park, Tom Kazimiers, Akira Fushiki, Ingrid V Andrade, Avinash Khandelwal, Javier Valdes-Aleman, Feng Li, Nadine Randel, Elizabeth Barsotti, Ana Correia, Richard D Fetter, Volker Hartenstein, Carey E Priebe, Joshua T Vogelstein, Albert Cardona, and Marta Zlatic. "The connectome of an insect brain" science, 2023. [URL]
- [98]
B. D. Pedigo, M. Winding, C. E. Priebe, and J. T. Vogelstein. "Bisected graph matching improves automated pairing of bilaterally homologous neurons from connectomes" Network Neuroscience, 2022. [URL]
- [97]
T. L. Athey, D. J. Tward, U. Mueller, Vogelstein Joshua T, and M. I. Miller. "Hidden Markov modeling for maximum probability neuron reconstruction" Communications Biology, 2022. [DOI]
- [96]
D. Kudithipudi, M. Aguilar-Simon, J. Babb, M. Bazhenov, D. Blackiston, J. Bongard, A. P. Brna, S. Chakravarthi Raja, N. Cheney, J. Clune, A. Daram, S. Fusi, P. Helfer, L. Kay, N. Ketz, Z. Kira, S. Kolouri, J. L. Krichmar, S. Kriegman, M. Levin, S. Madireddy, S. Manicka, A. Marjaninejad, B. McNaughton, R. Miikkulainen, Z. Navratilova, T. Pandit, A. Parker, P. K. Pilly, S. Risi, T. J. Sejnowski, A. Soltoggio, N. Soures, A. S. Tolias, D. Urbina-Meléndez, F. J. Valero-Cuevas, G. M. van de Ven, J. T. Vogelstein, F. Wang, R. Weiss, A. Yanguas-Gil, X. Zou, and H. Siegelmann. "Biological underpinnings for lifelong learning machines" Nature Machine Intelligence, (3)4:196-210, 2022. [DOI]
- [95]
S. Li, T. Jun, J. Tyler, E. Schadt, Y. Kao, Z. Wang, M. F. Konig, C. Bettegowda, J. T. Vogelstein, N. Papadopoulos, R. E. Parsons, R. Chen, E. E. Schadt, L. Li, and W. K. Oh. "Inpatient Administration of Alpha-1-Adrenergic Receptor Blocking Agents Reduces Mortality in Male COVID-19 Patients" Front. Med., 2022. [URL]
- [94]
J. Poline, D. N. Kennedy, F. T. Sommer, G. A. Ascoli, D. C. Van Essen, A. R. Ferguson, J. S. Grethe, M. J. Hawrylycz, P. M. Thompson, R. A. Poldrack, S. S. Ghosh, D. B. Keator, T. L. Athey, J. T. Vogelstein, H. S. Mayberg, and M. E. Martone. "Is Neuroscience FAIR? A Call for Collaborative Standardisation of Neuroscience Data" Neuroinformatics, 2022. [URL]
- [93]
J. Chung, B. Varjavand, J. Arroyo-Relión, A. Alyakin, J. Agterberg, M. Tang, C. E. Priebe, and J. T. Vogelstein. "Valid two-sample graph testing via optimal transport Procrustes and multiscale graph correlation with applications in connectomics" Stat, (1)11:e429, 2022.
- [92]
T. Hartung, L. Smirnova, I. E. M. Pantoja, A. Akwaboah, D. A. E. Din, C. Berlinicke, J. L. Boyd, B. S. Caffo, B. Cappiello, T. Cohen-Karni, L. Curley, R. Etienne-Cummings, R. Dastgheyb, D. H. Gracias, F. Gilbert, C. W. Habela, F. Han, T. Harris, K. Herrmann, E. J. Hill, Q. Huang, R. E. Jabbour, E. C. Johnson, B. J. Kagan, C. Krall, A. Levchenko, P. Locke, A. Maertens, M. Metea, A. R. Muotri, R. Parri, B. L. Paulhamus, J. D. Plotkin, P. Roach, J. C. Romero, J. C. Schwamborn, F. Sille, A. Szalay, K. Tsaioun, D. Tornero, J. T. Vogelstein, K. Wahlin, and D. J. Zack. "The Baltimore Declaration toward the exploration of organoid intelligence" Frontiers in Science, 2022.
- [91]
M. Powell, C. Clark, A. Alyakin, J. T. Vogelstein, and B. Hart. "Exploration of Residual Confounding in Analyses of Associations of Metformin Use and Outcomes in Adults With Type 2 Diabetes" JAMA Network Open, (11)5:e2241505–e2241505, 2022. [URL]
- [90]
V. Chandrashekhar, D. J. Tward, D. Crowley, A. K. Crow, M. A. Wright, B. Y. Hsueh, F. Gore, T. A. Machado, A. Branch, J. S. Rosenblum, K. Deisseroth, and J. T. Vogelstein. "CloudReg: automatic terabyte-scale cross-modal brain volume registration" Nature Methods, 2021. [DOI]
- [89]
M. Powell, A. Koenecke, J. Byrd, A. Nishimura, M. Konig, R. Xiong, S. Mahmood, V. B. Mucaj, L. Rose, S. Tamang, A. Sacarny, B. Caffo, S. Athey, E. Stuart, and J. Vogelstein. "Ten Rules for Conducting Retrospective Pharmacoepidemiological Analyses: Example COVID-19 Study" Frontiers in Pharmacology, 2021. [DOI]
- [88]
T. L. Athey, J. Teneggi, J. T. Vogelstein, D. Tward, U. Mueller, and M. I. Miller. "Fitting Splines to Axonal Arbors Quantifies Relationship between Branch Order and Geometry" Frontiers in Neuroinformatics, 2021. [URL]
- [87]
A. Koenecke, M. Powell, R. Xiong, Z. Shen, N. Fischer, S. Huq, A. M. Khalafallah, M. Trevisan, P. Sparen, J. J. Carrero, A. Nishimura, B. Caffo, E. A. Stuart, R. Bai, V. Staedtke, D. L. Thomas, N. Papadopoulos, K. W. Kinzler, B. Vogelstein, S. Zhou, C. Bettegowda, M. F. Konig, B. Mensh, J. T. Vogelstein, and S. Athey. ""Alpha-1 adrenergic receptor antagonists to prevent hyperinflammation and death from lower respiratory tract infection",journal=Elife" None, 2021. [DOI]
- [86]
C. Shen, S. Panda, and J. T. Vogelstein. "The Chi-Square Test of Distance Correlation" Journal of Computational and Graphical Statistics, (ja)0:1–21, 2021. [DOI]
- [85]
Ronan Perry, Gavin Mischler, Richard Guo, Theodore Lee, Alexander Chang, Arman Koul, Cameron Franz, Hugo Richard, Iain Carmichael, Pierre Ablin, Alexandre Gramfort, and Joshua T. Vogelstein. "mvlearn: Multiview Machine Learning in Python" Journal of Machine Learning Research, (109)22:1-7, 2021. [URL]
- [84]
J. T. Vogelstein, E. W. Bridgeford, M. Tang, D. Zheng, C. Douville, R. Burns, and M. Maggioni. "Supervised dimensionality reduction for big data" Nature Communications, (2872)12:1-9, 2021. [DOI]
- [83]
S. Li, T. Jun, Z. Wang, Y. Kao, E. Schadt, M. F. . B. Konig, J. T. Vogelstein, N. Papadopoulos, R. E. Parsons, and others. "COVID-19 outcomes among hospitalized men with or without exposure to alpha-1-adrenergic receptor blocking agents" Frontiers in Medicine, 2021. [URL]
- [82]
S. Wang, J. Arroyo, J. T. Vogelstein, and C. E. Priebe. "Joint Embedding of Graphs" Transactions on Pattern Analysis and Machine Intelligence, 2021. [URL]
- [81]
L. Rose, L. Graham, A. Koenecke, M. Powell, R. Xiong, Z. Shen, B. Mench, K. W. Kinzler, C. Bettegowda, B. Vogelstein, and others. "The association between Alpha-1 adrenergic receptor antagonists and in-hospital mortality from COVID-19" Frontiers in Medicine, 2021. [DOI]
- [80]
M. P. Milham, J. T. Vogelstein, and T. Xu. "Removing the Reliability Bottleneck in Functional Magnetic Resonance Imaging Research to Achieve Clinical Utility" JAMA Psychiatry, 2021. [DOI]
- [79]
J. Arroyo, A. Athreya, J. Cape, G. Chen, C. E. Priebe, and J. T. Vogelstein. "Inference for Multiple Heterogenous Networks with a Common Invariant Subspace" Journal of Machine Learning Research, (142)22:1-49, 2021. [URL]
- [78]
E. W. Bridgeford, S. Wang, Z. Wang, T. Xu, C. Craddock, J. Dey, G. Kiar, W. Gray-Roncal, C. Colantuoni, C. Douville, and others. "Eliminating accidental deviations to minimize generalization error and maximize replicability: Applications in connectomics and genomics" PLoS computational biology, (9)17:e1009279, 2021. [URL]
- [77]
R. M. Lawrence, E. W. Bridgeford, P. E. Myers, G. C. Arvapalli, S. C. Ramachandran, D. A. Pisner, P. F. Frank, A. D. Lemmer, A. Nikolaidis, and J. T. Vogelstein. "Standardizing human brain parcellations" Scientific data, (1)8:1–9, 2021. [URL]
- [76]
S. Hong, T. Xu, A. Nikolaidis, J. Smallwood, D. S. Margulies, B. Bernhardt, J. T. Vogelstein, and M. P. Milham. "Toward a connectivity gradient-based framework for reproducible biomarker discovery" NeuroImage, 2020. [DOI]
- [75]
Ting Xu, Karl-Heinz Nenning, Ernst Schwartz, Seok-Jun Hong, Joshua T. Vogelstein, Alexandros Goulas, Damien A. Fair, Charles E. Schroeder, Daniel S. Margulies, Jonny Smallwood, Michael P. Milham, and Georg Langs. "Cross-species functional alignment reveals evolutionary hierarchy within the connectome" NeuroImage, 2020. [DOI]
- [74]
J. W. Chow, A. Korchmaros, J. T. Vogelstein, M. P. Milham, and T. Xu. "Impact of concatenating fMRI data on reliability for functional connectomics" Neuroimage, 2020. [DOI]
- [73]
Karl-Heinz Nenning, Ting Xu, Ernst Schwartz, Jesus Arroyo, Adelheid Woehrer, Alexandre R. Franco, Joshua T. Vogelstein, Daniel S. Margulies, Hesheng Liu, Jonathan Smallwood, Michael P. Milham, and Georg Langs. "Joint embedding: A scalable alignment to compare individuals in a connectivity space" NeuroImage, 2020. [DOI]
- [72]
N. Wang, R. J. Anderson, D. G. Ashbrook, V. Gopalakrishnan, Y. Park, C. E. Priebe, Y. Qi, J. T. Vogelstein, R. W. Williams, and A. G. Johnson. "Variability and heritability of mouse brain structure: Microscopic MRI atlases and connectomes for diverse strains" NeuroImage (Cover Story), 2020. [DOI]
- [71]
M. A. Haendel, C. G. Chute, T. D. Bennett, D. A. Eichmann, J. Guinney, W. A. Kibbe, P. R. O. Payne, E. R. Pfaff, P. N. Robinson, J. H. Saltz, H. Spratt, C. Suver, J. Wilbanks, A. B. Wilcox, A. E. Williams, C. Wu, C. Blacketer, R. L. Bradford, J. J. Cimino, M. Clark, E. W. Colmenares, P. A. Francis, D. Gabriel, A. Graves, R. Hemadri, S. S. Hong, G. Hripscak, D. Jiao, J. G. Klann, K. Kostka, A. M. Lee, H. P. Lehmann, L. Lingrey, R. T. Miller, M. Morris, S. N. Murphy, K. Natarajan, M. B. Palchuk, U. Sheikh, H. Solbrig, S. Visweswaran, A. Walden, K. M. Walters, G. M. Weber, X. T. Zhang, R. L. Zhu, B. Amor, A. T. Girvin, A. Manna, N. Qureshi, M. G. Kurilla, S. G. Michael, L. M. Portilla, J. L. Rutter, C. P. Austin, and K. R. Gersing. "The National COVID Cohort Collaborative (N3C): Rationale, design, infrastructure, and deployment" Journal of the American Medical Informatics Association, 2020. [DOI]
- [70]
C. Shen and J. T. Vogelstein. "The exact equivalence of distance and kernel methods in hypothesis testing" AStA Advances in Statistical Analysis, 2020. [DOI]
- [69]
M. Madhyastha, G. Li, V. Strnadov-Neeley, J. Browne, J. T. Vogelstein, R. Burns, and C. E. Priebe. "Geodesic Forests" Proceedings of the 26th ACM SIGKDD International Conference on Knowledge Discovery and Data Mining, 2020. [DOI]
- [68]
M. Schulz, B. T. Yeo, J. T. Vogelstein, J. Mourao-Miranda, J. N. Kather, K. Kording, B. Richards, and D. Bzdok. "Different scalling of linear models and deep learning in UKBiobank brain images versus machine-learning datasets" Nat Commun, 2020. [DOI]
- [67]
M. Schulz, B. T. Yeo, J. T. Vogelstein, J. Mourao-Miranada, J. N. Kather, K. Kording, B. Richards, and D. Bzdok. "Different scaling of linear models and deep learning in UKBiobank brain images versus machine-learning datasets" Nat Commun, 2020. [DOI]
- [66]
Z. Wang, H. Sair, C. Crainiceanu, M. Lindquist, B. A. Landman, S. Resnick, J. T. Vogelstein, and B. S. Caffo. "On statistical tests of functional connectome fingerprinting" The Canadian Journal of Statistics, 2020. [DOI]
- [65]
A. S. Charles, B. Falk, N. Turner, T. D. Pereira, D. Tward, B. D. Pedigo, J. Chung, R. Burns, S. S. Ghosh, J. M. Kebschull, W. Silversmith, and J. T. Vogelstein. "Toward Community-Driven Big Open Brain Science: Open Big Data and Tools for Structure, Function, and Genetics" Annual Review of Neuroscience, (1)43:441-464, 2020. [DOI]
- [64]
Maximilian F. Konig, Mike Powell, Verena Staedtke, Ren-Yuan Bai, David L. Thomas, Nicole Fischer, Sakibul Huq, Adham M. Khalafallah, Allison Koenecke, Ruoxuan Xiong, Brett Mensh, Nickolas Papadopoulos, Kenneth W. Kinzler, Bert Vogelstein, Joshua T. Vogelstein, Susan Athey, Shibin Zhou, and Chetan Bettegowda. "Preventing cytokine storm syndrome in COVID-19 using alpha-1 adrenergic receptor antagonists" The Journal of Clinical Investigation, (7)130:3345-3347, 2020. [DOI]
- [63]
K. Mehta, R. F. Goldin, D. Marchette, J. T. Vogelstein, C. E. Priebe, and G. A. Ascoli. "Neuronal Classification from Network Connectivity via Adjacency Spectral Embedding" Network Neuroscience, 2020. [DOI]
- [62]
G. Franca, M. Rizzo, and J. T. Vogelstein. "Kernel k-Groups via Hartigan's Method" IEEE Transactions on Pattern Analysis and Machine Intelligence, 2020. [DOI]
- [61]
T. M. Tomita, J. Browne, C. Shen, J. Chung, J. L. Patsolic, B. Falk, J. Yim, C. E. Priebe, R. Burns, M. Maggioni, and J. T. Vogelstein. "Sparse Projection Oblique Randomer Forests" Journal of Machine Learninig Research, 2020. [URL]
- [60]
S. Hong, J. T. Vogelstein, A. Gozzi, B. C. Bernhardt, B. T. Yeo, M. P. Milham, and A. D. Martino. "Toward Neurosubtypes in Autism" Biological Psychiatry, (1)88:111 - 128, 2020. [DOI]
- [59]
A. Nikolaidis, A. S. Heinsfeld, T. Xu, P. Bellec, J. T. Vogelstein, and M. Milham. "Bagging Improves Reproducibility of Functional Parcellation of the Human Brain" NeuroImage, 2020. [URL]
- [58]
E. W. Bridgeford, S. Wang, Z. Yang, Z. Wang, . Xu, C. Craddock, G. Kiar, W. Gray-Roncal, C. E. Priebe, B. Caffo, M. Milham, X. Zuo, (CoRR), and J. T. Vogelstein. "Eliminating accidental deviations to minimize generalization error and maximize replicability: applications in connectomics and genomics" PLOS Computational Biology Methods, 2019. [URL]
- [57]
Y. Lee, C. Shen, C. E. Priebe, and J. T. Vogelstein. "Network dependence testing via diffusion maps and distance-based correlations" Biometrika, 2019. [DOI]
- [56]
R. Perry, T. M. Tomita, J. Patsolic, B. Falk, and J. T. Vogelstein. "Manifold Forests: Closing the Gap on Neural Networks" SIAM, 2019. [URL]
- [55]
J. Chung, B. D. Pedigo, E. W. Bridgeford, B. K. Varjavand, and J. T. Vogelstein. "GraSPy: Graph Statistics in Python" Journal of Machine Learning Research, (158)20:1–7, 2019. [URL]
- [54]
J. T. Vogelstein, E. W. Bridgeford, B. D. Pedigo, J. Chung, K. Levin, B. Mensh, and C. E. Priebe. "Connectal Coding: Discovering the Structures Linking Cognitive Phenotypes to Individual Histories" Current Opinion in Neurobiology, 2019. [DOI]
- [53]
C. E. Priebe, Y. Park, J. T. Vogelstein, J. M. Conroy, V. Lyzinski, M. Tang, A. Athreya, J. Cape, and E. Bridgeford. "On a two-truths phenomenon in spectral graph clustering" Proceedings of the National Academy of Sciences of the United States of America, (13)116:5995–6000, 2019. [DOI]
- [52]
J. J. Son, J. C. Clucas, C. White, A. Krishnakumar, J. T. Vogelstein, M. P. Milham, and A. Klein. "Thermal sensors improve wrist-worn position tracking" npj digital medicine, 2019. [DOI]
- [51]
H. Patsolic, S. Adali, J. T. Vogelstein, Y. . P. Park, G. Li, and V. Lyzinski. "Seeded Graph Matching Via Joint Optimization of Fidelity and Commensurability" ADS, astrophysics data system, 2019. [URL]
- [50]
J. T. Vogelstein, E. W. Bridgeford, Q. Wang, C. E. Priebe, M. Maggioni, and C. Shen. "Discovering and deciphering relationships across disparate data modalities" eLife, 2019. [DOI]
- [49]
R. Tang, M. Ketcha, A. Badea, E. D. Calabrese, D. S. Margulies, J. T. Vogelstein, C. E. Priebe, and D. L. Sussman. "Connectome Smoothing via Low-rank Approximations" Transactions in Medical Imaging, 2018. [URL]
- [48]
J. T. Vogelstein, E. Bridgeford, M. Tang, D. Zheng, R. Burns, and M. Maggioni. "Supervised dimensionality reduction for big data" Nature Communications, 2018. [URL]
- [47]
C. Shen, C. E. Priebe, and J. T. Vogelstein. "From Distance Correlation to Multiscale Graph Correlation" Journal of the American Statistical Association, 2018. [URL]
- [46]
J. T. Vogelstein, E. Perlman, B. Falk, A. Baden, W. Gray Roncal, V. Chandrashekhar, F. Collman, S. Seshamani, J. L. Patsolic, K. Lillaney, M. Kazhdan, R. Hider, D. Pryor, J. Matelsky, T. Gion, P. Manavalan, B. Wester, M. Chevillet, E. T. Trautman, K. Khairy, E. Bridgeford, D. M. Kleissas, D. J. Tward, A. K. Crow, B. Hsueh, M. A. Wright, M. I. Miller, S. J. Smith, R. J. Vogelstein, K. Deisseroth, and R. Burns. "A Community-Developed Open-Source Computational Ecosystem for Big Neuro Data" Nature Methods, (11)15:846–847, 2018. [DOI]
- [45]
A. Athreya, D. E. Fishkind, M. Tang, C. E. Priebe, Y. Park, J. T. Vogelstein, K. Levin, V. Lyzinski, Y. Qin, and D. L. Sussman. "Statistical Inference on Random Dot Product Graphs: a Survey" Journal of Machine Learning Research, 2018. [URL]
- [44]
J. D. Cohen, L. Li, Y. Wang, C. Thoburn, B. Afsari, L. Danilova, C. Douville, A. A. Javed, F. Wong, A. Mattox, R. H. Hruban, C. L. Wolfgang, M. G. Goggins, M. D. Molin, T. L. Wang, R. Roden, A. P. Klein, J. Ptak, L. Dobbyn, J. Schaefer, N. Silliman, M. Popoli, J. T. Vogelstein, J. D. Browne, R. E. Schoen, R. E. Brand, J. Tie, P. Gibbs, H. L. Wong, A. S. Mansfield, J. Jen, S. M. Hanash, M. Falconi, P. J. Allen, S. Zhou, C. Bettegowda, L. A. Diaz, C. Tomasetti, K. W. Kinzler, B. Vogelstein, A. M. Lennon, and N. Papadopoulos. "Detection and localization of surgically resectable cancers with a multi-analyte blood test" Science, (6378)359:926–930, 2018. [DOI]
- [43]
E. L. Dyer, W. G. Roncal, H. L. Fernandes, D. Gürsoy, V. De Andrade, R. Vescovi, K. Fezzaa, X. Xiao, J. T. Vogelstein, C. Jacobsen, K. P. Körding, and N. Kasthuri. "Quantifying Mesoscale Neuroanatomy Using X-Ray Microtomography" eNeuro, 2017. [DOI]
- [42]
D. Durante, D. B. Dunson, and J. T. Vogelstein. "Rejoinder: Nonparametric Bayes Modeling of Populations of Networks" Journal of the American Statistical Association, 2017. [DOI]
- [41]
D. Durante, D. B. Dunson, and J. T. Vogelstein. "Nonparametric Bayes Modeling of Populations of Networks" Journal of the American Statistical Association, (520)112:1516–1530, 2017. [DOI]
- [40]
G. Kiar, K. J. Gorgolewski, D. Kleissas, W. G. Roncal, B. Litt, B. Wandell, R. A. Poldrack, M. Wiener, R. J. Vogelstein, R. Burns, and J. T. Vogelstein. "Science in the cloud (SIC): A use case in MRI connectomics" GigaScience, (5)6:1–10, 2017. [DOI]
- [39]
N. Binkiewicz, J. T. Vogelstein, and K. Rohe. "Covariate-assisted spectral clustering" Biometrika, (2)104:361–377, 2017. [DOI]
- [38]
S. Chen, K. Liu, Y. Yang, Y. Xu, S. Lee, M. Lindquist, B. S. Caffo, and J. T. Vogelstein. "An M-estimator for reduced-rank system identification" Pattern Recognition Letters, 2017. [DOI]
- [37]
D. G. C. Hildebrand, M. Cicconet, R. M. Torres, W. Choi, T. M. Quan, J. Moon, A. W. Wetzel, A. Scott Champion, B. J. Graham, O. Randlett, G. S. Plummer, R. Portugues, I. H. Bianco, S. Saalfeld, A. D. Baden, K. Lillaney, R. Burns, J. T. Vogelstein, A. F. Schier, W. C. A. Lee, W. K. Jeong, J. W. Lichtman, and F. Engert. "Whole-brain serial-section electron microscopy in larval zebrafish" Nature, (7654)545:345–349, 2017. [DOI]
- [36]
C. Shen, J. T. Vogelstein, and C. E. Priebe. "Manifold matching using shortest-path distance and joint neighborhood selection" Pattern Recognition Letters, 2017. [DOI]
- [35]
A. K. Simhal, C. Aguerrebere, F. Collman, J. T. Vogelstein, K. D. Micheva, R. J. Weinberg, S. J. Smith, and G. Sapiro. "Probabilistic fluorescence-based synapse detection" PLoS Computational Biology, 2017. [DOI]
- [34]
Q. Wang, M. Zhang, T. Tomita, J. T. Vogelstein, S. Zhou, N. Papadopoulos, K. W. Kinzler, and B. Vogelstein. "Selected reaction monitoring approach for validating peptide biomarkers" Proceedings of the National Academy of Sciences of the United States of America, (51)114:13519–13524, 2017. [DOI]
- [33]
D. Zheng, D. Mhembere, V. Lyzinski, J. T. Vogelstein, C. E. Priebe, and R. Burns. "Semi-external memory sparse matrix multiplication for billion-node graphs" IEEE Transactions on Parallel and Distributed Systems, (5)28:1470–1483, 2017. [DOI]
- [32]
L. Chen, C. Shen, J. T. Vogelstein, and C. E. Priebe. "Robust Vertex Classification" IEEE Transactions on Pattern Analysis and Machine Intelligence, (3)38:578–590, 2016. [DOI]
- [31]
D. Koutra, N. Shah, J. T. Vogelstein, B. Gallagher, and C. Faloutsos. "DeltaCon: Principled Massive-Graph Similarity Function with Attribution" ACM Transactions on Knowledge Discovery from Data, 2016. [DOI]
- [30]
V. Lyzinski, D. E. Fishkind, M. Fiori, J. T. Vogelstein, C. E. Priebe, and G. Sapiro. "Graph Matching: Relax at Your Own Risk" IEEE Transactions on Pattern Analysis and Machine Intelligence, (1)38:60–73, 2016. [DOI]
- [29]
R. D. Airan, J. T. Vogelstein, J. J. Pillai, B. Caffo, J. J. Pekar, and H. I. Sair. "Factors affecting characterization and localization of interindividual differences in functional connectivity using MRI" Human Brain Mapping, (5)37:1986–1997, 2016. [DOI]
- [28]
C. E. Priebe, D. L. Sussman, M. Tang, and J. T. Vogelstein. "Statistical Inference on Errorfully Observed Graphs" Journal of Computational and Graphical Statistics, (4)24:930–953, 2015. [DOI]
- [27]
L. Chen, J. T. Vogelstein, V. Lyzinski, and C. E. Priebe. "A Joint Graph Inference Case Study: the C.elegans Chemical and Electrical Connectomes" Worm, 2015. [DOI]
- [26]
W. R. Gray Roncal, D. M. Kleissas, J. T. Vogelstein, P. Manavalan, K. Lillaney, M. Pekala, R. Burns, R. J. Vogelstein, C. E. Priebe, M. A. Chevillet, and G. D. Hager. "An automated images-to-graphs framework for high resolution connectomics" Frontiers in Neuroinformatics, 2015. [DOI]
- [25]
K. M. Harris, J. Spacek, M. E. Bell, P. H. Parker, L. F. Lindsey, A. D. Baden, J. T. Vogelstein, and R. Burns. "A resource from 3D electron microscopy of hippocampal neuropil for user training and tool development" Scientific Data, 2015. [DOI]
- [24]
N. Kasthuri, K. J. Hayworth, D. R. Berger, R. L. Schalek, J. A. Conchello, S. Knowles-Barley, D. Lee, A. Vázquez-Reina, V. Kaynig, T. R. Jones, M. Roberts, J. L. Morgan, J. C. Tapia, H. S. Seung, W. G. Roncal, J. T. Vogelstein, R. Burns, D. L. Sussman, C. E. Priebe, H. Pfister, and J. W. Lichtman. "Saturated Reconstruction of a Volume of Neocortex" Cell, (3)162:648–661, 2015. [DOI]
- [23]
V. Lyzinski, D. L. Sussman, D. E. Fishkind, H. Pao, L. Chen, J. T. Vogelstein, Y. Park, and C. E. Priebe. "Spectral clustering for divide-and-conquer graph matching" Parallel Computing, 2015. [DOI]
- [22]
J. T. Vogelstein, J. M. Conroy, V. Lyzinski, L. J. Podrazik, S. G. Kratzer, E. T. Harley, D. E. Fishkind, R. J. Vogelstein, and C. E. Priebe. "Fast Approximate Quadratic programming for graph matching" PLoS ONE, 2015. [DOI]
- [21]
J. T. Vogelstein and C. E. Priebe. "Shuffled Graph Classification: Theory and Connectome Applications" Journal of Classification, (1)32:3–20, 2015. [DOI]
- [20]
D. E. Carlson, J. T. Vogelstein, Q. Wu, W. Lian, M. Zhou, C. R. Stoetzner, D. Kipke, D. Weber, D. B. Dunson, and L. Carin. "Multichannel Electrophysiological Spike Sorting via Joint Dictionary Learning and Mixture Modeling" IEEE Transactions on Biomedical Engineering, (1)61:41–54, 2014. [DOI]
- [19]
E. M. Sweeney, J. T. Vogelstein, J. L. Cuzzocreo, P. A. Calabresi, D. S. Reich, C. M. Crainiceanu, and R. T. Shinohara. "A comparison of supervised machine learning algorithms and feature vectors for MS lesion segmentation using multimodal structural MRI" PLoS ONE, 2014. [DOI]
- [18]
J. T. Vogelstein, Y. Park, T. Ohyama, R. A. Kerr, J. W. Truman, C. E. Priebe, and M. Zlatic. "Discovery of brainwide neural-behavioral maps via multiscale unsupervised structure learning" Science, (6182)344:386–392, 2014. [DOI]
- [17]
N. C. Weiler, F. Collman, J. T. Vogelstein, R. Burns, and S. J. Smith. "Synaptic molecular imaging in spared and deprived columns of mouse barrel cortex with array tomography" Scientific Data, 2014. [DOI]
- [16]
R. C. Craddock, S. Jbabdi, C. G. Yan, J. T. Vogelstein, F. X. Castellanos, A. Di Martino, C. Kelly, K. Heberlein, S. Colcombe, and M. P. Milham. "Imaging human connectomes at the macroscale" Nature Methods, (6)10:524–539, 2013. [DOI]
- [15]
C. E. Priebe, J. Vogelstein, and D. Bock. "Optimizing the quantity/quality trade-off in connectome inference" Communications in Statistics - Theory and Methods, (19)42:3455–3462, 2013. [DOI]
- [14]
J. T. Vogelstein, W. G. Roncal, R. J. Vogelstein, and C. E. Priebe. "Graph classification using signal-subgraphs: Applications in statistical connectomics" IEEE Transactions on Pattern Analysis and Machine Intelligence, (7)35:1539–1551, 2013. [DOI]
- [13]
D. Dai, H. He, J. T. Vogelstein, and Z. Hou. "Accurate prediction of AD patients using cortical thickness networks" Machine Vision and Applications, (7)24:1445–1457, 2012. [DOI]
- [12]
D. E. Fishkind, D. L. Sussman, M. Tang, J. T. Vogelstein, and C. E. Priebe. "Consistent adjacency-spectral partitioning for the stochastic block model when the model parameters are unknown" SIAM Journal on Matrix Analysis and Applications, (1)34:23–39, 2012. [DOI]
- [11]
W. R. Gray, J. A. Bogovic, J. T. Vogelstein, B. A. Landman, J. L. Prince, and R. J. Vogelstein. "Magnetic Resonance Connectome Automated Pipeline: An Overview" IEEE Pulse, (2)3:42–48, 2012. [DOI]
- [10]
N. J. Roberts, J. T. Vogelstein, G. Parmigiani, K. W. Kinzler, B. Vogelstein, and V. E. Velculescu. "The predictive capacity of personal genome sequencing" Science Translational Medicine, 2012. [DOI]
- [9]
S. B. Hofer, H. Ko, B. Pichler, J. Vogelstein, H. Ros, H. Zeng, E. Lein, N. A. Lesica, and T. D. Mrsic-Flogel. "Differential connectivity and response dynamics of excitatory and inhibitory neurons in visual cortex" Nature Neuroscience, (8)14:1045–1052, 2011. [DOI]
- [8]
Y. Mishchencko, J. T. Vogelstein, and L. Paninski. "A Bayesian approach for inferring neuronal conectivity from calcium fluorescent imaging data" The annals of applied statistics, 2011. [DOI]
- [7]
J. T. Vogelstein, R. J. Vogelstein, and C. E. Priebe. "Are mental properties supervenient on brain properties?" Scientific Reports, 2011. [DOI]
- [6]
L. Paninski, Y. Ahmadian, D. G. Ferreira, S. Koyama, K. Rahnama Rad, M. Vidne, J. Vogelstein, and W. Wu. "A new look at state-space models for neural data" Journal of Computational Neuroscience, (1-2)29:107–126, 2009. [DOI]
- [5]
J. T. Vogelstein, A. M. Packer, T. A. Machado, T. Sippy, B. Babadi, R. Yuste, and L. Paninski. "Fast non-negative deconvolution for spike train inference from population calcium imaging" Journal of Neurophysiology, 2009. [DOI]
- [4]
J. T. Vogelstein, B. O. Watson, A. M. Packer, R. Yuste, B. Jedynak, and L. Paninski. "Spike inference from calcium imaging using sequential Monte Carlo methods" Biophysical Journal, (2)97:636–655, 2009. [DOI]
- [3]
R. J. Vogelstein, U. Mallik, J. T. Vogelstein, and G. Cauwenberghs. "Dynamically reconfigurable silicon array of spiking neurons with conductance-based synapses" IEEE Transactions on Neural Networks, (1)18:253–265, 2007. [DOI]
- [2]
J. T. Vogelstein, L. H. Snyder, and D. E. Angelaki. "Accuracy of saccades to remembered targets as a function of body orientation in space" Journal of Neurophysiology, (1)90:521–524, 2003. [DOI]
- [1]
D. L. Greenspan, D. C. Connolly, R. Wu, R. Y. Lei, J. T. Vogelstein, Y. T. Kim, J. E. Mok, N. Muñoz, F. X. Bosch, K. Shah, and K. R. Cho. "Loss of FHIT expression in cervical carcinoma cell lines and primary tumors" Cancer Research, 1997. [URL]
Manuscripts Not Yet Accepted
- [12]
S. Panda, C. Shen, R. Perry, J. Zorn, A. Lutz, C. E. Priebe, and J. T. Vogelstein. "Universally Consistent K-sample Tests via Dependence Measures" Statistics \& Probability Letters, 2025. [DOI]
- [11]
K. Konishcheva, B. Leventhal, M. Koyama, S. Panda, J. T. Vogelstein, M. Milham, A. Lindner, and A. Klein. "Accurate and efficient data-driven psychiatric assessment using machine learning" PsyArXiv, 2024. [URL]
- [10]
J. Chung, E. W. Bridgeford, M. Powell, D. Pisner, T. Xu, and J. T. Vogelstein. "Are human connectomes heritable?" bioRxiv, 2024. [URL]
- [9]
C. Shen, S. Panda, and J. T. Vogelstein. "Learning Interpretable Characteristic Kernels via Decision Forests" arXiv, 2023. [URL]
- [8]
Eric W. Bridgeford, Jaewon Chung, Brian Gilbert, Sambit Panda, Adam Li, Cencheng Shen, Alexandra Badea, Brian Caffo, and Joshua T. Vogelstein. "Learning sources of variability from high-dimensional observational studies" arXiv, 2023. [URL]
- [7]
J. Dey, W. LeVine, H. Xu, A. De Silva, T. M. Tomita, A. Geisa, T. Chu, J. Desman, and J. T. Vogelstein. "Deep Discriminative to Kernel Generative Networks for In- and Out-of-distribution Calibrated Inference" arXiv, 2022. [URL]
- [6]
T. Xu, J. Cho, G. Kiar, E. W. Bridgeford, J. T. Vogelstein, and M. P. Milham. "A Guide for Quantifying and Optimizing Measurement Reliability for the Study of Individual Differences" bioRxiv, 2022. [URL]
- [5]
Haoyin Xu, Jayanta Dey, Sambit Panda, and Joshua T. Vogelstein. "Simplest Streaming Trees" arXiv, 2021. [URL]
- [4]
Haoyin Xu, Kaleab A. Kinfu, Will LeVine, Sambit Panda, Jayanta Dey, Michael Ainsworth and Yu-Chung Peng, Madi Kusmanov, Florian Engert, Christopher M. White, Joshua T. Vogelstein, and Carey E. Priebe. "When are Deep Networks really better than Decision Forests at small sample sizes, and how?" arXiv, 2021. [URL]
- [3]
S. Panda, S. Palaniappan, J. Xiong, E. W. Bridgeford, . Mehta, C. Shen, and J. T. Vogelstein. "hyppo: A Multivariate Hypothesis Testing Python Package" arXiv, 2021. [URL]
- [2]
V. Gopalakrishnan, J. Chung, E. Bridgeford, B. D. Pedigo, J. Arroyo, L. Upchurch, G. A. Johnsom, N. Wang, Y. Park, C. E. Priebe, and J. T. Vogelstein. "Discovery of Multi-Level Network Differences Across Populations of Heterogeneous Connectomes" arXiv, 2020. [URL]
- [1]
J. T. Vogelstein, J. Dey, H. S. Helm, W. LeVine, Mehta, Ronak D, T. M. Tomita, H. Xu, A. Geisa, Q. Wang, . M. van de Ven, and others. "A Simple Lifelong Learning Approach" arXiv, 2020. [URL]
Conference Papers
- [27]
A. De Silva, R. Ramesh, R. Yang*, S. Yu, J. T. Vogelstein, and P. Chaudhari. "Prospective Learning: Learning for a Dynamic Future" Advances in neural information processing systems, 2024. [URL]
- [26]
Q. Wang, M. A. Powell, A. Geisa, E. Bridgeford, C. E. Priebe, and J. T. Vogelstein. "Why do networks have inhibitory/negative connections?" Proceedings of the IEEE/CVF International Conference on Computer Vision (ICCV), 2023. [URL]
- [25]
A. De Silva, R. Ramesh, C. Priebe, P. Chaudhari, and J. T. Vogelstein. "The value of out-of-distribution data" International Conference on Machine Learning, 2023. [URL]
- [24]
Q. Wang, Michael A. Powell, Ali Geisa, Eric W. Bridgeford, and Joshua T. Vogelstein. "Polarity is all you need to learn and transfer faster" Proceedings of the 40th International Conference on Machine Learning, 2023. [URL]
- [23]
J. T. Vogelstein, T. Verstynen, K. P. Kording, L. Isik, J. W. Krakauer, R. Etienne-Cummings, E. L. Ogburn, C. E. Priebe, R. Burns, K. Kutten, J. J. Knierim, J. B. Potash, T. Hartung, L. Smirnova, P. Worley, A. Savonenko, I. Phillips, M. I. Miller, R. Vidal, J. Sulam, A. Charles, N. J. Cowan, M. Bichuch, A. Venkataraman, C. Li, N. Thakor, J. M. Kebschull, M. Albert, J. Xu, M. H. Shuler, B. Caffo, T. Ratnanather, A. Geisa, S. Roh, E. Yezerets, M. Madhyastha, J. J. How, T. M. Tomita, J. Dey, N. Huang, J. M. Shin, K. A. Kinfu, P. Chaudhari, B. Baker, A. Schapiro, D. Jayaraman, E. Eaton, M. Platt, L. Ungar, L. Wehbe, A. Kepecs, A. Christensen, O. Osuagwu, B. Brunton, B. Mensh, A. R. Muotri, G. Silva, F. Puppo, F. Engert, E. Hillman, J. Brown, C. White, and W. Yang. "Prospective Learning: Back to the Future" 2nd Conference on Lifelong Learning Agents, 2023, 2022. [URL]
- [22]
M. Madhyastha, K. Lillaney, J. Browne, J. T. Vogelstein, and R. Burns. "BLOCKSET (Block-Aligned Serialized Trees): Reducing Inference Latency for Tree Ensemble Deployment" Proceedings of the 27th ACM SIGKDD Conference on Knowledge Discovery and Data Mining, 2021. [DOI]
- [21]
M. Madhyastha, G. Li, V. Strnadová-Neeley, J. Browne, J. T. Vogelstein, R. Burns, and C. E. Priebe. "Geodesic Forests" Proceedings of the 26th ACM SIGKDD International Conference on Knowledge Discovery and Data Mining, 2020. [DOI]
- [20]
A. Nikolaidis, A. S. Heinsfeld, T. Xu, P. Bellec, J. Vogelstein, and M. Milham. "Bagging Improves Reproducibility of Functional Parcellation of the Human Brain" bioRxiv, 2019. [DOI]
- [19]
J. Browne, D. Mhembere, T. M. Tomita, J. T. Vogelstein, and R. Burns. "Forest packing: Fast Parallel, Decision Forests" SIAM International Conference on Data Mining, SDM, 2018. [DOI]
- [18]
K. Lillaney, D. Kleissas, A. Eusman, E. Perlman, W. Gray Roncal, J. T. Vogelstein, and R. Burns. "Building NDStore through hierarchical storage management and microservice processing" Proceedings - IEEE 14th International Conference on eScience, e-Science, 2018. [DOI]
- [17]
D. Zheng, D. Mhembere, J. T. Vogelstein, C. E. Priebe, and R. Burns. "FlashR: R-Programmed Parallel and Scalable Machine Learning using SSDs" PPoPP, 2017. [URL]
- [16]
K. S. Kutten, N. Charon, M. I. Miller, J. T. Ratnanather, J. Matelsky, A. D. Baden, K. Lillaney, K. Deisseroth, L. Ye, and J. T. Vogelstein. "A large deformation diffeomorphic approach to registration of CLARITY images via mutual information" Lecture Notes in Computer Science (including subseries Lecture Notes in Artificial Intelligence and Lecture Notes in Bioinformatics), 2017. [DOI]
- [15]
D. Mhembere, C. E. Priebe, J. T. Vogelstein, and R. Burns. "knor : A NUMA-Optimized In-Memory , Distributed and Semi-External-Memory k-means Library" Proceedings of the 26th International Symposium on High-Performance Parallel and Distributed Computing, 2017. [URL]
- [14]
T. M. Tomita, M. Maggioni, and J. T. Vogelstein. "ROFLMAO: Robust oblique forests with linear MAtrix operations" Proceedings of the 17th SIAM International Conference on Data Mining, SDM 2017, 2017. [DOI]
- [13]
K. S. Kutten, J. T. Vogelstein, N. Charon, L. Ye, K. Deisseroth, and M. I. Miller. "Deformably registering and annotating whole CLARITY brains to an atlas via masked LDDMM" Optics, Photonics and Digital Technologies for Imaging Applications IV, 2016. [DOI]
- [12]
W. G. Roncal, M. Pekala, V. Kaynig-Fittkau, D. M. Kleissas, J. T. Vogelstein, H. Pfister, R. Burns, R. J. Vogelstein, M. A. Chevillet, and G. D. Hager. "VESICLE: Volumetric Evaluation of Synaptic Inferfaces using Computer Vision at Large Scale" British Machine Vision Conference, 2015. [DOI]
- [11]
D. Zheng, D. Mhembere, R. Burns, J. T. Vogelstein, C. E. Priebe, and A. S. Szalay. "FlashGraph: Processing Billion-Node Graphs on an Array of Commodity SSDs" USENIX Conference on File and Storage Technologies, 2015. [DOI]
- [10]
D. Mhembere, W. Gray Roncal, D. Sussman, C. E. Priebe, R. Jung, S. Ryman, R. J. Vogelstein, J. T. Vogelstein, and R. Burns. "Computing scalable multivariate glocal invariants of large (brain-) graphs" 2013 IEEE Global Conference on Signal and Information Processing, GlobalSIP 2013 - Proceedings, 2013. [DOI]
- [9]
W. G. Roncal, Z. H. Koterba, D. Mhembere, D. M. Kleissas, J. T. Vogelstein, R. Burns, A. R. Bowles, D. K. Donavos, S. Ryman, R. E. Jung, L. Wu, V. Calhoun, and R. J. Vogelstein. "MIGRAINE: MRI graph reliability analysis and inference for connectomics" 2013 IEEE Global Conference on Signal and Information Processing, 2013. [DOI]
- [8]
R. Burns, W. G. Roncal, D. Kleissas, K. Lillaney, P. Manavalan, E. Perlman, D. R. Berger, D. D. Bock, K. Chung, L. Grosenick, N. Kasthuri, N. C. Weiler, K. Deisseroth, M. Kazhdan, J. Lichtman, R. C. Reid, S. J. Smith, A. S. Szalay, J. T. Vogelstein, and R. J. Vogelstein. "The Open Connectome Project Data Cluster: Scalable Analysis and Vision for High-Throughput Neuroscience" ACM International Conference Proceeding Series, 2013. [DOI]
- [7]
D. E. Carlson, V. Rao, J. T. Vogelstein, and L. Carin. "Real-Time Inference for a Gamma Process Model of Neural Spiking" Advances in Neural Information Processing Systems 26, 2013. [URL]
- [6]
B. Cornelis, Y. Yang, J. T. Vogelstein, A. Dooms, I. Daubechies, and D. Dunson. "Bayesian crack detection in ultra high resolution multimodal images of paintings" 18th International Conference on Digital Signal Processing, 2013. [DOI]
- [5]
M. Fiori, P. Sprechmann, J. Vogelstein, P. Muse, and G. Sapiro. "Robust Multimodal Graph Matching: Sparse Coding Meets Graph Matching" Advances in Neural Information Processing Systems, 2013. [URL]
- [4]
D. Koutra, J. T. Vogelstein, and C. Faloutsos. "DELTACON: A principled massive-graph similarity function" Proceedings of the 2013 SIAM International Conference on Data Mining, SDM 2013, 2013. [DOI]
- [3]
V. Kulkarni, J. S. Pudipeddi, L. Akoglu, J. T. Vogelstein, R. J. Vogelstein, S. Ryman, and R. E. Jung. "Sex differences in the human connectome" Brain and Health Informatics, 2013. [DOI]
- [2]
F. Petralia, J. Vogelstein, and D. B. Dunson. "Multiscale Dictionary Learning for Estimating Conditional Distributions" Advances in Neural Information Processing Systems, 2013. [URL]
- [1]
Q. J. Huys, J. Vogelstein, and P. Dayan. "Psychiatry: Insights into depression through normative decision-making models" Advances in Neural Information Processing Systems, 2008. [URL]
Book Chapters
- [1]
J. T. Vogelstein, R. Vogelstein, and C. E. Priebe. "A Neurocognitive Graph-Theoretical Approach to Understanding the Relationship Between Minds and Brains" CSHL conference on Neural Circuits, 2010.
Technical Reports
- [30]
T. L. Athey, M. A. Wright, M. Pavlovic, V. Chandrashekhar, K. Deisseroth, M. I. Miller, and J. T. Vogelstein. "BrainLine: An Open Pipeline for Connectivity Analysis of Heterogeneous Whole-Brain Fluorescence Volumes" Neuroinformatics, 2023. [URL]
- [29]
V. Chandrashekhar, D. J. Tward, D. Crowley, A. K. Crow, M. A. Wright, B. Y. Hsueh, F. Gore, T. A. Machado, A. Branch, J. S. Rosenblum, K. Deisseroth, and J. T. Vogelstein. "CloudReg: automatic terabyte-scale cross-modal brain volume registration" Nature Methods, 2021. [DOI]
- [28]
Ronan Perry, Ronak Mehta, Richard Guo, Eva Yezerets, Jesús Arroyo, Mike Powell and Hayden Helm, Cencheng Shen, and Joshua T. Vogelstein. "Random Forests for Adaptive Nearest Neighbor Estimation of Information-Theoretic Quantities" arXiv, 2021. [URL]
- [27]
Ali Saad-Eldin, Benjamin D. Pedigo, Carey E. Priebe, and Joshua T. Vogelstein. "Graph Matching via Optimal Transport" arXiv, 2021. [URL]
- [26]
Jayanta Dey, Ali Geisa, Ronak Mehta, Tyler M. Tomita, Hayden S. Helm, Eric Eaton, Jeffery Dick, Carey E. Priebe, and Joshua T. Vogelstein. "Towards a theory of out-of-distribution learning" arXiv, 2021. [URL]
- [25]
H. S. Helm, R. D. Mehta, B. Duderstadt, W. Yang, C. M. White, A. Geisa, J. T. Vogelstein, and C. E. Priebe. "A partition-based similarity for classification distributions" arXiv, 2020. [URL]
- [24]
Jaewon Chung, Eric Bridgeford, Jesus Arroyo, Benjamin D. Pedigo, Ali Saad-Eldin, Vivek Gopalakrishnan, Liang Xiang, Carey E. Priebe, and Joshua Vogelstein. "Statistical Connectomics" arXiv, 2020. [URL]
- [23]
J. T. Vogelstein. "P-Values in a Post-Truth World" arXiv, 2020. [URL]
- [22]
H. S. Helm, A. Basu, A. Athreya, Y. Park, J. T. Vogelstein, M. Winding, M. Zlatic, A. Cardona, P. Bourke, J. Larson, C. White, and C. E. Priebe. "Learning to rank via combining representations" arXiv, 2020. [URL]
- [21]
C. E. Priebe, J. T. Vogelstein, F. Engert, and C. M. White. "Modern Machine Learning: Partition Vote" bioRxiv, 2020. [DOI]
- [20]
Polina Golland, Jack Gallant, Greg Hager, Hanspeter Pfister, Christos Papadimitriou, Stefan Schaal, and Joshua T. Vogelstein. "A New Age of Computing and the Brain" arXiv, 2020. [URL]
- [19]
Tyler M. Tomita and Joshua T. Vogelstein. "Robust Similarity and Distance Learning via Decision Forests" arXiv, 2020. [URL]
- [18]
D. Mhembere, D. Zheng, J. T. Vogelstein, C. E. Priebe, and R. Burns. "Graphyti: A Semi-External Memory Graph Library for FlashGraph" arXiv, 2019. [URL]
- [17]
H. Helm, J. V. Vogelstein, and C. E. Priebe. "Vertex Classification on Weighted Networks" arXiv, 2019. [URL]
- [16]
J. Xiong, C. Shen, J. Arroyo, and J. T. Vogelstein. "Graph Independence Testing" arXiv, 2019. [URL]
- [15]
D. Mhembere, D. Zheng, C. E. Priebe, J. T. Vogelstein, and R. Burns. "clusterNOR: A NUMA-Optimized Clustering Framework" arxiv, 2019. [URL]
- [14]
A. Branch, D. Tward, J. T. Vogelstein, Z. Wu, and M. Gallagher. "An optimized protocol for iDISCO+ rat brain clearing, imaging, and analysis" bioRxiv, 2019. [DOI]
- [13]
D. S. Greenberg, D. J. Wallace, K. Voit, S. Wuertenberger, U. Czubayko, A. Monsees, T. Handa, J. T. Vogelstein, R. Seifert, Y. Groemping, and J. N. Kerr. "Accurate action potential inference from a calcium sensor protein through biophysical modeling" bioRxiv, 2018. [DOI]
- [12]
G. Kiar, E. Bridgeford, W. G. Roncal, (CoRR), V. Chandrashekhar, D. Mhembere, S. Ryman, X. Zuo, D. S. Marguiles, R. C. Craddock, C. E. Priebe, R. Jung, V. Calhoun, B. Caffo, R. Burns, M. P. Milham, and J. Vogelstein. "A High-Throughput Pipeline Identifies Robust Connectomes But Troublesome Variability" bioRxiv, 2018. [DOI]
- [11]
G. Kiar, R. J. Anderson, A. Baden, A. Badea, E. W. Bridgeford, A. Champion, V. Chandrashekhar, F. Collman, B. Duderstadt, A. C. Evans, F. Engert, B. Falk, T. Glatard, W. R. G. Roncal, D. N. Kennedy, J. Maitin-Shepard, R. A. Marren, O. Nnaemeka, E. Perlman, S. Seshamani, E. T. Trautman, D. J. Tward, P. A. Valdés-Sosa, Q. Wang, M. I. Miller, R. Burns, and J. T. Vogelstein. "NeuroStorm: Accelerating Brain Science Discovery in the Cloud" arXiv, 2018. [URL]
- [10]
S. Wang, C. Shen, A. Badea, C. E. Priebe, and J. T. Vogelstein. "Signal Subgraph Estimation Via Vertex Screening" arXiv, 2018. [URL]
- [9]
G. Kiar, E. Bridgeford, V. Chandrashekhar, D. Mhembere, R. Burns, W. R. G. Roncal, and J. T. Vogelstein. "A comprehensive cloud framework for accurate and reliable human connectome estimation and meganalysis" bioRxiv, 2017. [URL]
- [8]
R. Tang, M. Tang, J. T. Vogelstein, and C. E. Priebe. "Robust Estimation from Multiple Graphs under Gross Error Contamination" arXiv, 2017. [URL]
- [7]
C. E. Priebe, Y. Park, M. Tang, A. Athreya, V. Lyzinski, J. T. Vogelstein, Y. Qin, B. Cocanougher, K. Eichler, M. Zlatic, and A. Cardona. "Semiparametric spectral modeling of the Drosophila connectome" arXiv, 2017. [URL]
- [6]
D. Zheng, D. Mhembere, J. T. Vogelstein, C. E. Priebe, and R. Burns. "FlashR: R-Programmed Parallel and Scalable Machine Learning using SSDs" CoRR, abs/1604.06414, 2017. [URL]
- [5]
D. Zheng, R. Burns, J. Vogelstein, C. E. Priebe, and A. S. Szalay. "An SSD-based eigensolver for spectral analysis on billion-node graphs" arXiv, 2016. [URL]
- [4]
D. Zheng, D. Mhembere, J. T. Vogelstein, C. E. Priebe, and R. Burns. "Flashmatrix: parallel, scalable data analysis with generalized matrix operations using commodity ssds" arXiv, 2016. [URL]
- [3]
A. Sinha, W. Roncal, and N. Kasthuri. "Automatic Annotation of Axoplasmic Reticula in Pursuit of Connectomes" arXiv, 2014. [URL]
- [2]
M. Kazhdan, R. Burns, B. Kasthuri, J. Lichtman, J. Vogelstein, and J. Vogelstein. "Gradient-Domain Processing for Large EM Image Stacks" arXiv, 2013. [URL]
- [1]
A. Banerjee, J. Vogelstein, and D. Dunson. "Parallel inversion of huge covariance matrices" arXiv, 2013. [URL]
Other Publications
- [11]
Ashwin De Silva, Rahul Ramesh, Carey E. Priebe, Pratik Chaudhari, and Joshua T. Vogelstein. "The Value of Out-of-Distribution Data" Workshop on Out-of-Distribution Generalization in Computer Vision, European Conference on Computer Vision, 2022. [URL]
- [10]
E. W. Bridgeford, D. Sussman, V. Lyzinski, Y. Qin, Y. Park, B. Caffo, C. E. Priebe, and J. T. Vogelstein. "What is Connectome Coding?" SfN 2018 course book, 2018. [URL]
- [9]
J. Caplis and J. T. Vogelstein. "Glass box vs. black box" Pensions Investments, 2017.
- [8]
J. T. Vogelstein, K. Amunts, A. Andreou, D. Angelaki, G. A. Ascoli, C. Bargmann, R. Burns, C. Cali, F. Chance, G. Church, H. Cline, T. Coleman, D. Stephanie de La Rochefoucauld, A. B. Elgoyhen, R. E. Cummings, A. Evans, K. Harris, M. Hausser, S. Hill, S. Inverso, C. Jackson, V. Jain, R. Kass, B. Kasthuri, A. Kepecs, G. Kiar, K. Kording, S. P. Koushika, J. Krakauer, S. Landis, J. Layton, Q. Luo, A. Marblestone, D. Markowitz, J. McArthur, B. Mensh, M. P. Milham, P. Mitra, P. Neskovic, M. Nicolelis, R. O'Brien, A. Oliva, G. Orban, H. Peng, E. Perlman, M. Picciotto, M. Poo, J. Poline, A. Pouget, S. Raghavachari, J. Roskams, A. P. Schaffer, T. Sejnowski, F. T. Sommer, N. Spruston, L. Swanson, A. Toga, R. J. Vogelstein, A. Zador, R. Huganir, and M. I. Miller. "Grand challenges for global brain sciences" F1000Research, 2016. [DOI]
- [7]
J. T. Vogelstein, B. Mensh, M. Häusser, N. Spruston, A. C. Evans, K. Kording, K. Amunts, C. Ebell, J. Muller, M. Telefont, S. Hill, S. P. Koushika, C. Calì, P. A. Valdés-Sosa, P. B. Littlewood, C. Koch, S. Saalfeld, A. Kepecs, H. Peng, Y. O. Halchenko, G. Kiar, M. M. Poo, J. B. Poline, M. P. Milham, A. P. Schaffer, R. Gidron, H. Okano, V. D. Calhoun, M. Chun, D. M. Kleissas, R. J. Vogelstein, E. Perlman, R. Burns, R. Huganir, and M. I. Miller. "To the Cloud! A Grassroots Proposal to Accelerate Brain Science Discovery" Neuron, (3)92:622–627, 2016. [DOI]
- [6]
R. Burns, J. T. Vogelstein, and A. S. Szalay. "From cosmos to connectomes: The evolution of data-intensive science" Neuron, (6)83:1249–1252, 2014. [DOI]
- [5]
P. Golland, J. Gallant, G. Hager, H. Pfister, C. Papadimitriou, S. Schaal, and J. T. Vogelstein. "A New Age of Computing and the Brain: Report of the CCC Brain Workshop" CCC Brain Workshop, 2014. [URL]
- [4]
V. Vogelstein and J. T. "Q and A: What is the Open Connectome Project?" Neural Systems and Circuits, 2011. [DOI]
- [3]
R. Yuste, J. MacLean, J. Vogelstein, and L. Paninski. "Imaging action potentials with calcium indicators" Cold Spring Harbor Protocols, (8)6:985–989, 2011. [DOI]
- [2]
V. Vogelstein and J. T. "Oopsi: a family of optimal optical spike inference algorithms for inferring neural connectivity from population calcium imaging" Learning, 2009. [URL]
- [1]
J. T. Vogelstein, J. V. Vogelstein, and B. Vogelstein. "NIH Grant Application Testing the effects of genetic variations using MINIME technology" Science, (5448)286:2300–2301, 1999. [DOI]
Funding
External Research Support: Current
- 2022-2027 NIH, The Heart and the Mind: An Integrative Approach to Brain-Body Interactions in the Zebrafish 2U19NS104653
PI: F. Engert
Role on Project: Co-Investigator
Term: 01-Sep-2022 to 31-Aug-2024
Funding to lab, entire period: $412,500 (total)
Funding to lab, current year:Johns Hopkins University will be responsible for developing all algorithms and software in support of the Atlas project, as well as running the Data Core. This will include writing software to store, manage, and visualize the data, as well as algorithms for scalable analysis and support of modeling.
- 2020-2024 NSF, Neural Net Learning for Graph NSF 2113099
PI: C. Shen
Role on Project: Co-Investigator
Term: 01-Sep-2021 to 31-Aug-2024
Funding to lab, entire period: $73,597 (total)
Funding to lab, current year:Goal of this project is methodological development, theoretical investigation, and simulation and real data experimentation toward the end goal of principled understanding and advancement of the mathematics and science of graph neural network
- 2021-2024 NIH, An Alignment Framework for Mapping Brain Dynamics and Substrates of Human Cognition Across Species 1RF1MH128696
PI: T. Xu
Role on Project: Co-Investigator
Term: 01-Sep-2021 to 31-Aug-2024
Funding to lab, entire period: $270,189 (total)
Funding to lab, current year:We will continue collecting, organizing, and analyzing another cohort of the NKI-Rockland Sample.
- 2021-2027 NIH, The NKI Rockland Sample II: An Open Resource of Multimodal Brain, Physiology \& Behavior Data from a Community Lifespan Sample 2U19NS104653
PI: M. Milham
Role on Project: Co-Investigator
Term: 01-Jul-2022 to 30-Apr-2026
Funding to lab, entire period: $3,831,854 (total)
Funding to lab, current year:The major goal is to establish multimodal MRI and electrophysiology lifespan sample to open and prospectively share with the larger scientific community.
- 2020-2025 NSF, Collaborative Research: Transferable, Hierarchical, Expensive, Optimal, Robust, Interpretable Networks NSF 20-540
PI: R. Vidal
Role on Project: Co-Investigator
Term: 01-Sep-2020 to 31-Aug-2025
Funding to lab, entire period: $1,650,000 (direct)
Funding to lab, current year: $660,000 (direct)The goal of this project is to develop a mathematical, statistical and computational frame- work that helps explain the success of current network arcitectures, understand its pitfalls, and guide the design of novel architectures with guaranteed confidence, robustness, inter- pretability, optimality, and transferability
- 2020 -- Microsoft, Federated Causal Inference for Multi-site Real-World Evidence \& Clinical Trial Analysis Studies in Pandemic Preparedness
PI: M. Powell
Role on Project: Co-Investigator
Term: 01-Aug-2020 to current
Funding to lab, entire period: N/A
Funding to lab, current year: N/AThis project will conduct federated retrospective analyses designed to assess the benefit of off-label drug use by pooling multiple disparate databases, to help prioritize and guide subsequent initiation and recruitment of randomized clinical trials. This will include evaluating the impact of the target drugs on patient outcomes from diseases similar to COVID-19, such as pneumonia or acute respiratory distress, generating artificial datasets using generative adversarial networks to asses performance of methods when 'ground truth' is known, applying the best methods to analyze the effect of the target drugs on the outcomes of COVID-19 patients across hospital systems, and using the results to evaluate the potential of these drugs and suggest guidelines for clinical trials.
- 2020-2023 NIH, Graspy: A python package for rigorous statistical analysis of populations of attributed connectomes NIH MH-19-147
PI: J. Vogelstein
Role on Project: Principal Investigator
Term: 01-Jul-2020 to 30-Jun-2023
Funding to lab, entire period: $861,240 (direct) $1,416,279 (total)
Funding to lab, current year: $283,301 (direct) $471,082 (total)The goal of this project is to establish a state-of-the-art toolbox for analysis of connectomes, spanning taxa, scale, and complexity. we will develop and extend implementations to enable neurobiologists to (1) estimate latent structure from attributed connectomes, (2) identify meaningful clusters among populations of connectomes, and (3) detect relationships be- tween connectomes and multivariate phenotypes
- 2020-2025 NSF, NeuroNex2: Enabling Identification and Impact of Synaptic Weight in Functional Networks NSF 2014862
PI: K. Harris
Role on Project: Co-Investigator
Term: 01-Apr-2020 to 31-Mar-2025
Funding to lab, entire period: $609,294 (direct) $997,719 (total)
Funding to lab, current year: $121,587 (direct) $199,543 (total)The goal is to develop the requisite technology to understand the impact of synaptic weight on functional networks
- 2020-2025 NSF, CAREER: Foundational Statistical Theory and Methods for Analysis of Populations of Attributed Connectomes NSF 17-537
PI: J. Vogelstein
Role on Project: Principal Investigator
Term: 01-Jan-2020 to 31-Dec-2025
Funding to lab, entire period: $630,230 (total) $384,873 (direct)
Funding to lab, current year: $126,046 (total) $76,975 (direct)The goal is to establish foundaitonal theory and methods for analyzing populations of attributed connectomes
- 2019-2023 NIH, Brain Networks in Mouse Models of Aging NIH RO1AG066184-01
PI: A. Badea
Role on Project: Co-Investigator
Term: 01-Dec-2019 to 30-Nov-2023
Funding to lab, entire period: N/A
Funding to lab, current year: $205,998The goal of this grant is to generate connectomes and RNA-seq transcriptomes to characterize and differentiate APOE mice as a model of aging
- 2019 -- Microsoft, Microsoft Research Award
PI: J. Vogelstein
Role on Project: Principal Investigator
Term: Unrestricted Gift
Funding to lab, entire period: $50,000 (total)
Funding to lab, current year: N/AResearch and development of neuroscience and connectomes around neuronal circuit and system modeling, application of time-series-of-graphs and dynamics to neuronal signaling analysis and connectomes, and in the abstractions of matter, math, machines that point toward complex systems composed of low-level components
External Research Support: Completed
- 2020-2022 NSF, AI Institute: Planning: BI4ALL: Understanding Biological NSF 20-503
PI: K. Kording
Role on Project: Co-Investigator
Term: 01-Oct-2020 to 31-Jul-2022
Funding to lab, entire period: N/A
Funding to lab, current year: \$79,629 (direct)The goal of this project is to plan an AI institution via several meetings and workshops
- 2019-2022 NIH, Accessible technologies for high-throughput, whole-brain reconstructions of molecularly characterized mammalian neurons NIH RFA-MH-19-148
PI: M. Muller
Role on Project: Co-Investigator
Term: 01-Sep-2019 to 31-Aug-2022
Funding to lab, entire period: \$1,180,445 (total) \$753,974 (direct)
Funding to lab, current year: \$383,482 (total) \$251,325 (direct)The overall goal of the proposal is to develop technologies for the brain wide reconstruction of axonal arbors of molecularly defined neurons. The proposal aims at overcoming barriers in neuronal labeling, imaging and computation to achieve this goal, and to develop a technology platform that can be scaled to all neurons of the brain
- 2019-2020 NIH, Reproducible imaging-based brain growth charts for psychiatry NIH R01MH120482-01
PI: T. Satterthwaite
Role on Project: Co-Investigator
Term: 01-Aug-2019 to 31-May-2020
Funding to lab, entire period: \$362,861 (total) \$231,276 (direct)
Funding to lab, current year: N/AAggregate, harmonize, and analyze existing large-scale pediatric neuroimaging datasets to identify normative and clinical brain growth curves
- 2018 -- 2021 NSF, SemiSynBio: Collaborative Research: YeastOns: Neural Networks Implemented in Communication Yeast Cells NSF 1807369
PI: E. Schulman
Role on Project: Co-Investigator
Term: 16-Jul-2018 to 30-Jun-2021
Funding to lab, entire period: \$263,942 (total) \$172,971 (direct)
Funding to lab, current year: \$87,980 (total) \$57,657 (direct)Provide neuroscience and machine learning expertise to guide the design of the computa- tional learning capabilities of the system
- 2018 -- 2020 Schmidt Science Foundation, Connectome Coding at the Synaptic Scale Nascent Innovation Grant 128503
PI: J. Vogelstein
Role on Project: Principal Investigator
Term: 01-Jan-2018 to 31-Dec-2020
Funding to lab, entire period: \$250,000 (total)
Funding to lab, current year: N/AStudy learning and plasticity at an unprecedented scale, revealing the dynamics of large populations of synapses comprising an entire local cortical circuit. No previously conducted experiment could answer the questions about the dynamics of large populations of synapses, which is crucial to understanding the learning process
- 2017 -- 2021 DARPA, Continual Learning Across Synapses, Circuits, and Brain Areas FA8650-18-2-7834
PI: A. Tolias
Role on Project: Co-Investigator
Term: 01-Nov-2017 to 30-Oct-2021
Funding to lab, entire period: \$796,715 (total) \$486,666 (direct)
Funding to lab, current year: \$199,179 (total) \$121,667 (direct)Develop the pre-processing analysis pipeline for the imaging data collected in this project
- 2017 -- 2021 DARPA, Lifelong Learning Forests FA8650-18-2-7834
PI: J. Vogelstein
Role on Project: Principal Investigator
Term: 01-Nov-2017 to 31-Oct-2021
Funding to lab, entire period: \$1,839,308 (total) \$1,123,474 (direct)
Funding to lab, current year: \$199,179 (total) \$121,667 (direct)Lifelong Learning Forests (L2Fs) will learn continuously, selectively adapting to new environ- ments and circumstances utilizing top-down feedback to impact low-level processing, with provable statistical guarantees, while maintaining computational tractability at scale
- 2017 -- 2022 NIH, Sensorimotor processing, decision making, and internal states: towards a realistic multiscale circuit model of the larval zebrafish brain NIH 1U19NS104653-01
PI: F. Engert
Role on Project: Co-Investigator
Term: 01-Sep-2017 to 31-Aug-2022
Funding to lab, entire period: \$1,050,000 (total) \$655,206 (direct) (JHU sub-award)
Funding to lab, current year: \$210,000 (total) \$131,041 (direct)Generate a realistic multiscale circuit model of the larval zebrafish’s brain – the multiscale virtual fish (MSVF). The model will span spatial ranges from the nanoscale at the synaptic level, to local microcircuits to inter-area connectivity - and its ultimate purpose is to explain and simulate the quantitative and qualitative nature of behavioral output across various timescales
- 2017 -- 2020 NSF, NeuroNex Innovation Award: Towards Automatic Analysis of Multi-Terabyte Cleared Brains NSF 1707298
PI: J. Vogelstein
Role on Project: Principal Investigator
Term: 01-Sep-2017 to 31-Aug-2020 (No Cost Extension)
Funding to lab, entire period: \$959,999 (total) \$588,758 (direct)
Funding to lab, current year: \$320,000 (total) \$196,252 (direct)We propose to lower the barrier to connecting data to analyses and models by providing a coherent cloud computational ecosystem that minimizes current bottlenecks in the scientific process
- 2017 -- 2020 NIH, CRCNS US-German Res Prop: functional computational anatomy of the auditory cortex NIH 1R01DC016784-01
PI: J. MRatnanather
Role on Project: Co-Investigator
Term: 01-Jul-2017 to 30-Jun-2020
Funding to lab, entire period: \$747,143 (total) \$458,519 (direct)
Funding to lab, current year: N/ACreate a robust computational framework for analyzing the cortical ribbon in a specific region: the auditory cortex
- 2017 -- 2020 NSF, Multiscale Generalized Correlation: A Unified Distance-Based Correlation Measure for Dependence Discovery NSF 1921310
PI: S. Cencheng
Role on Project: Co-Investigator
Term: 01-May-2017 to 30-Apr-2020
Funding to lab, entire period: \$200,000 (total) \$124,189 (direct)
Funding to lab, current year: N/AEstablish a unified methodology framework for statistical testing in high-dimensional, noisy, big data, through theoretical advancements, comprehensive simulations, and real data experiments
- 2017 -- 2019 NSF, NeuroNex Technology Hub: Towards the International Brain Station for Accelerating and Democratizing Neuroscience Data Analysis and Modeling NSF 16-569
PI: J. Vogelstein
Role on Project: Principal Investigator
Term: 2017 to 2019
Funding to lab, entire period: \$246,773
Funding to lab, current year: N/AWe propose to lower the barrier to connecting data to analyses and models by providing a coherent cloud computational ecosystem that minimizes current bottlenecks in the scientific process
- 2017 -- 2018 The Kavli Foundation, The International Brain Station 90071826
PI: J. Vogelstein
Role on Project: Principal Investigator
Term: 2017 to 2018
Funding to lab, entire period: \$50,000 (total) \$50,000 (direct)
Funding to lab, current year: N/ATake the first few steps towards building the international brain station
- 2017 -- 2018 NSF, Brain Comp Infra: EAGER: BrainLab CI: Collaborative, Community Experiments ACI-1649880
PI: B. Miller
Role on Project: Co-Investigator
Term: 2017 to 2018
Funding to lab, entire period: \$294,599 (total) \$180,736 (direct)
Funding to lab, current year: N/AThe BrainLab CI prototype system will deploy an experimental-management infrastruc- ture that allows users to construct community-wide experiments that implement data and metadata controls on the inclusion and exclusion of data
- 2017 -- 2018 DARPA, The Brain Ark 90076467
PI: J. Vogelstein
Role on Project: Principal Investigator
Term: 2017 to 2018
Funding to lab, entire period: \$92,376 (total) \$56,499.08 (direct)
Funding to lab, current year: N/ACharacterize the statistical properties of the individual graphs, to identify circuit motifs, both that specialize in a species specific fashion, and that are preserved across species. As a test, will compare the connectomes of sea lions and coyotes
- 2016 -- 2020 DARPA, D3M: What Would Tukey Do? FA8750-17-2-0112
PI: C. Priebe
Role on Project: Co-Investigator
Term: 01-Oct-2016 to 30-Sep-2020
Funding to lab, entire period: \$4,406,360 (total) \$2,746,050 (direct)
Funding to lab, current year: N/ADevelop theory and methods for generating a discoverable archive of data modeling primi- tives and for automatically selecting model primitives and for composing selected primitives into complex modeling pipelines based on user-specified data and outcome(s) of interest
- 2016 -- 2019 NSF, A Scientific Planning Workshop for Coordinating Brain Research Around the Globe NIH RFA-MH-19-148
PI: J. Vogelstein
Role on Project: Principal Investigator
Term: 2016 to 2019
Funding to lab, entire period: \$97,950 (total) \$97,950 (direct)
Funding to lab, current year:This travel grant is for the expressed purposes of gathering researchers from around the globe to discuss the new way to further brain research during part one of a two day conference
- 2016 -- 2019 NSF, A Scientific Planning Workshop for Coordinating Brain Research Around the Globe NSF 1637376
PI: J. Vogelstein
Role on Project: Principal Investigator
Term: 2016 to 2019
Funding to lab, entire period: \$16,327 (total) \$14,491 (direct)
Funding to lab, current year: N/AThis travel grant is for the expressed purposes of gathering researchers from around the globe to further discuss advancements in brain research during the second part of a two day conference
- 2015 -- 2018 DARPA, From RAGs to Riches: Utilizing Richly Attributed Graphs to Reason from N66001-15-C-40401
PI: J. Vogelstein
Role on Project: Principal Investigator
Term: 01-Sep-2019 to 31-Aug-2022
Funding to lab, entire period: \$2,103,091.60 (total) \$1,298,204 (direct)
Funding to lab, current year: N/AMultiple, large, multifarious brain imaging datasets are rapidly becoming standards in neuroscience. Yet, we lack the tools to analyze individual datasets, much less populations thereof. Therefore, we will develop theory and methods to analyze and otherwise make such data available
- 2014 -- 2016 DARPA, Scalable Grain Graph Analyses Using Big-Memory, High-IPS Compute Architectures N66001-14-1-4028
PI: R. Burns
Role on Project: Co-Investigator
Term: 2014 to 2016
Funding to lab, entire period: \$39,882 (total) \$28,272 (direct)
Funding to lab, current year: N/ABuild software infrastructure to enable analytics on billion node, terabyte sized networks using commodity hardware
- 2014 -- 2019 NIH, Synaptomes of Mouse and Man NIH R01NS092474
PI: S. Smith
Role on Project: Co-Investigator
Term: 2014 to 2019
Funding to lab, entire period: \$756,417 (total) \$491,341 (direct)
Funding to lab, current year: N/AThe major goals of this project are to discover the synaptic diversity and complexity in mammalian brains, specifically comparing and contrasting humans with mice, the leading experimental animal
- 2012 -- 2015 National Institute of Biomedical Imaging and Bioengineering, CRCNS: Data Sharing: The EM open Connectome Project RO1EB16411
PI: R. Burns
Role on Project: Co-Investigator
Term: 2012 to 2015
Funding to lab, entire period: \$70,823 (total) \$46,517 (direct)
Funding to lab, current year: N/ADevelop cyberinfrastructure to support management, visualization, storage, and analysis of large-scale electron microscopy data
Invited Talks
- [58]
J. T. Vogelstein. "It's about time: Learning for a Dynamic World" MATRIX AI Consortium for Human Well-Being, University of Texas San Antonio, USA, 2025.
- [57]
J. T. Vogelstein. "Learning for a Dynamic Future" Kavli NDI, Johns Hopkins University, USA, 2025.
- [56]
J. T. Vogelstein. "Learing when the future is partially predictable" University of Washington, Seattle, WA, USA, 2024.
- [55]
J. T. Vogelstein. "Learing when the future is partially predictable" Allen Institute for Brain Science, Seattle, WA, USA, 2024.
- [54]
J. T. Vogelstein. "Prospective Learning: Learning for a dynamic future" Cold Spring Harbor Laboratory, NY, USA, 2024.
- [53]
J. T. Vogelstein. "Moderator, SNF Global Center Third Expert Gathering: Consensus Building for Scalable Biometric Tools and Measures to Facilitate Global Mental Health Research" Child Mind Institute, NY, USA, 2024.
- [52]
J. T. Vogelstein. "Fixing Artificial Intelligence via Natural Intelligence (YouTube) " Nemertes Next, Royal Sonesta Harbor Court, Baltimore, MD, USA, 2024.
- [51]
J. T. Vogelstein. "Learning the way that animals leaned to learn" Schizophrenia Center, Johns Hopkins University, Baltimore, MD, USA, 2024.
- [50]
J. T. Vogelstein. "Surprise! IID++ Out of Distribution & Prospective Learning" Simons Foundation, New York, NY, 2023.
- [49]
B. D. Pedigo. "Hypothesis testing for connectome comparisons: a statistical analysis of bilateral symmetry in an insect brain connectome" Drexel University, Philadelphia, PA, 2022.
- [48]
Ali Geisa. "Towards a theory of out-of-distribution learning" JHU BME, Baltimore, MD, USA, 2021.
- [47]
E. Bridgeford. "Eliminating Accidental Deviations in Human Connectomics" JHU BME, Baltimore, MD, USA, 2021.
- [46]
J. Chung. "Heritablity of Human Structural Connectomes" JHU BME, Baltimore, MD, USA, 2021.
- [45]
J. Dey. "Omnidirectional Lifelong Learning via Ensembling Representations" JHU BME, Baltimore, MD, USA, 2021.
- [44]
J. T. Vogelstein. "FIRM Guiding Principles for scientific software development and stewardship" JHU BME, Baltimore, MD, USA, 2021.
- [43]
J. T. Vogelstein. "Jovo++" JHU BME, Baltimore, MD, USA, 2021.
- [42]
J. T. Vogelstein. "Reality Transurfing: Chapter 1" JHU BME, Baltimore, MD, USA, 2021.
- [41]
J. T. Vogelstein. "Lifelong Learning: Theory and Practice" Darpa L2M PI Meeting, 2021.
- [40]
J. T. Vogelstein. "Lifelong Learning and Beyond" Darpa L2M PI Meeting, 2021.
- [39]
J. T. Vogelstein. "Lifelong Learning: Theory and Context" Darpa L2M PI Meeting, 2021.
- [38]
J. T. Vogelstein. "Lifelong Learning: Theory and Practice and Coresets" Darpa L2M PI Meeting, 2021.
- [37]
J. T. Vogelstein. "Lifelong Learning" North Carolina State University, Raleigh, NC, USA, 2020.
- [36]
J. T. Vogelstein. "Lifelong Learning" Morgan State University, Baltimore, MD, USA, 2020.
- [35]
J. T. Vogelstein. "Lifelong Learning: Moving Beyond Avoiding Catastrophic Forgetting" Johns Hopkins Mathematical Institute for Data Science, Baltimore, MD, USA, 2020.
- [34]
J. T. Vogelstein. "Open Access to the Brain: a Computer "Connectome" Links Brain Images in Fine Detail" JHM Boot Camp, Baltimore, MD, USA, 2019.
- [33]
J. T. Vogelstein. "Big Biomedical Data Science" Sol Goldman International Conference, Baltimore, MD, USA, 2019.
- [32]
J. T. Vogelstein. "Journey to Here" JHU BMES talks, Baltimore, MD, USA, 2019.
- [31]
J. T. Vogelstein. "NeuroData (Science)" Kavli, Baltimore, MD, USA, 2019.
- [30]
J. T. Vogelstein. "NeuroData Tools" NeuroData Hackashop, Baltimore, MD, USA, 2019.
- [29]
J. T. Vogelstein. "Biomedical Big Data and Data Science" JHU BME, Baltimore, MD, USA, 2019.
- [28]
J. T. Vogelstein. "Data Intensive Brain Science" Kavli Neuroscience Discovery Institute, Baltimore, MD, USA, 2018.
- [27]
J. T. Vogelstein. "Using Big Data Science to Understand What Goes On in our Heads" SOHOP Faculty Spotlight, Baltimore, MD, USA, 2018.
- [26]
J. T. Vogelstein. "Engineering the Future of Medicine: Data Intensive Biomedical Science" Johns Hopkins University Biomedical Engineering, Baltimore, MD, USA, 2018.
- [25]
J. T. Vogelstein. "Data Coordination and Data Resources for the BRAIN Initiative" 4th Annual BRAIN Initiative Investigators Meeting, Rockville, MD, USA, 2018.
- [24]
J. T. Vogelstein. "The International Brain Station (TIBS)" JHU BME and Tsinghua University, Baltimore, MD, USA, 2017.
- [23]
J. T. Vogelstein. "Using Big Data Science to Understand What Goes on in Our Heads" SOHOP Faculty Spotlight, Baltimore, MD, USA, 2017.
- [22]
J. T. Vogelstein. "Challenges and Opportunities in Big Data for Neuroscientists" Society for Neuroscience: DC Metro Area Chapter Keynote Address, Washington, DC, USA, 2017.
- [21]
J. T. Vogelstein. "Opportunities and Challenges in Big Data Neuroscience" Society for Neuroscience, Washington D.C., USA, 2017.
- [20]
J. T. Vogelstein. "NeuroStorm" Global Brain Workshop 2 JHU, Baltimore, MD, USA, 2017.
- [19]
J. T. Vogelstein. "The International Brain Station (TIBS)" United Nations Global Brain Workshop Meeting, Baltimore, MD, USA, 2016.
- [18]
J. T. Vogelstein. "Using Big Data Science to Understand What Goes on in Our Heads" SOHOP Faculty Spotlight, Baltimore, MD, USA, 2016.
- [17]
J. T. Vogelstein. "The International Brain Station (TIBS)" Kavli Foundation, Baltimore, MD, USA, 2016.
- [16]
J. T. Vogelstein. "NeuroData 2016" NeuroData Lab Retreat, 2016.
- [15]
J. T. Vogelstein. "Global Brain Workshop 2016" Global Brain Workshop NSF+JHU at Kavli, Baltimore, MD, USA, 2016.
- [14]
J. T. Vogelstein. "Global Brain Workshop 2016" Kavli Neuroscience Discovery Institute & Center for Imaging Science, Baltimore, MD, USA, 2016.
- [13]
J. T. Vogelstein. "Learning a Data-Driven Nosology:Progress, Challenges & Opportunities" Kavli Neuroscience Discovery Institute & Center for Imaging Science, Baltimore, MD, USA, 2016.
- [12]
J. T. Vogelstein. "NeuroData:Enabling Terascale Neuroscience" Kavli Neuroscience Discovery Institute & Center for Imaging Science, Baltimore, MD, USA, 2016.
- [11]
J. T. Vogelstein. "NeuroData:Enabling Terascale Neuroscience" JHU Kavli Neuroscience Discovery Institute, Baltimore, MD, USA, 2016.
- [10]
J. T. Vogelstein, M. I. Miller, and R. Hunganir. "Global Brain Workshop 2016" Kavli Neuroscience Discovery Institute & Center for Imaging Science @ JHU, Baltimore, MD, USA, 2016.
- [9]
J. T. Vogelstein. "Special Symposium: Neuroscience in the 21st Century" Kavli, Baltimore, MD, USA, 2015.
- [8]
J. T. Vogelstein. "Open Connectome Project: Lowering the Barrier to Entry of Big Data Neuroscience" Institute for Computational Medicine at Johns Hopkins University, Baltimore, MD, USA, 2015.
- [7]
J. T. Vogelstein. "Open Source Platform for Heterogenous Brain Data" figshare, 2015.
- [6]
J. T. Vogelstein. "Big (Neuro) Statistics" Kavli Salon, Chicago, IL, USA, 2014.
- [5]
J. T. Vogelstein. "Open-Science Platform for Heterogeneous Brain Data: Opportunities and Challenges" Kavli, Baltimore, MD, USA, 2014.
- [4]
J. T. Vogelstein. "Big (Neuro) Statistics" Kavli Salon, Baltimore, MD, USA, 2014.
- [3]
J. T. Vogelstein. "Decision Theoretic Approach to Statistical Inference" Guest Lecture in Current Topics in Machine Learning, Johns Hopkins University, Baltimore, MD, USA, 2012.
- [2]
J. T. Vogelstein. "Once we get connectomes, what the \%\#* are we going to do with them?" Institute of Neuroinformatics, Boston, MA, USA, 2011.
- [1]
J. T. Vogelstein. "Inferring spike times given typical time-series fluorescence observations" Department of Applied Mathematics and Statistics, Johns Hopkins University, Baltimore, MD, USA, 2008.
Other Talks
- [80]
J. T. Vogelstein. "Learning: the next frontier" National University of Public Service, Budapest, Hungary, 2024.
- [79]
J. T. Vogelstein. "Learning the way that animals leaned to learn" Department of Biomedical Engineering, McGill University, Canada, 2024.
- [78]
J. T. Vogelstein. "Probably Approximately Correct in the Future" Banff International Research Station for Mathematical Innovation and Discovery, Oaxaca, Mexico, 2024.
- [77]
Qingyang Wang. "Why do networks need negative weights?" None, 2022.
- [76]
E. Bridgeford. "Simulating a Realization of a Stochastic Block Model" ABCD-ReproNim Program, 2022.
- [75]
E. Bridgeford. "Community Detection and Model Selection in SBMs" ABCD-ReproNim Program, 2022.
- [74]
S. Panda, C. Shen, and J. T. Vogelstein. "Nonparametric MANOVA via Independence Testing" Global Young Scientists Summit, 2022.
- [73]
B. D. Pedigo, M. Winding, M. Zlatic, A. Cardona, C. E. Priebe, and J. T. Vogelstein. "Maggot brain, mirror image? A statistical analysis of bilateral symmetry in an insect brain connectome" Neuromatch 4.0, 2021.
- [72]
B. D. Pedigo and J. T. Vogelstein. "graspologic: A python package for rigorous statistical analysis of populations of attributed connectomes" BRAIN Informatics Webinar, 2021.
- [71]
S. Panda, C. Shen, and J. T. Vogelstein. "Nonparametric MANOVA via Independence Testing" BRAIN Initiative Meeting, 2021.
- [70]
B. D. Pedigo. "Network data science for bilateral brains: Applications in the larval Drosophila connectome" NIH & DOE Brain Connectivity Workshop Series, 2021.
- [69]
J. T. Vogelstein. "OOD DARPA Presentation" DARPA, 2021.
- [68]
J. T. Vogelstein. "Lifelong Learning and Beyond" DARPA L2M, 2021.
- [67]
J. Vogelstein. "The role of the connectome in achieving artificial general intelligence" Yale School of Medicine, Whistler Scientific Workshop, Whistler, BC, Canada, 2020.
- [66]
J. Vogelstein. "Lifelong Learning via Ensembling General Representations" None, 2020.
- [65]
H. Helm, R. Mehta, C. E. Priebe, R. Arora, and J. T. Vogelstein. "A Theory and Practice of Lifelong Learnable Forest" Kavli Neural Systems Institute, Rockefeller University, New York City, NY, USA, 2020.
- [64]
J. T. Vogelstein. "Lifelong Learning" Columbia University, New York City, NY, USA, 2020.
- [63]
J. T. Vogelstein. "Ailey in an Hour: (A "Soup-to-Nuts" Pipeline for Analysis of Whole Cleared Brain Data)" NeuroNex, Cornell University, Ithaca, NY, USA, 2019.
- [62]
J. T. Vogelstein, H. Helm, R. Mehta, C. E. Priebe, and R. Arora. "A Theory and Practice of the Lifelong Learnable" L2M, 2019.
- [61]
J. T. Vogelstein and R. Burns. "Data Science Core" Harvard University, Carmridge, MA, USA, 2019.
- [60]
J. Chung. "Statistical Methods for Population of Connectomes" Organization of Human Brain Mapping, Rome, Italy, 2019.
- [59]
J. Browne. "Forest Packing: Fast Parallel, Decision Forests" SIAM International Conference on Data Mining, Calgary, Alberta, Canada, 2019.
- [58]
D. Tward. "Brain mapping tools for neuroscience research" NeuroNex, Cornell University, Ithaca, NY, USA, 2019.
- [57]
J. T. Vogelstein. "Big Data and the Life Sciences" Sloan Foundation, New York City, NY, USA, 2019.
- [56]
J. T. Vogelstein. "Statistical Foundations For Connectomics" Max Planck / HHMI Connectomics Meeting, Berlin, Germany, 2019.
- [55]
J. T. Vogelstein. "Connectal Coding" Dipy Workshop, Bloomington, Indiana, USA, 2019.
- [54]
J. T. Vogelstein. "Lifelong Learning Forests" L2M, 2019.
- [53]
J. T. Vogelstein. "Connectome Coding" Society for Neuroscience, San Diego, CA, USA, 2018.
- [52]
J. T. Vogelstein. "NeuroData: A Community-developed open-source computational ecosystem for big neuro data" NeuroNex, Cornell University, Ithaca, NY, USA, 2018.
- [51]
J. T. Vogelstein. "A Community-Developed Open-Source Computational Ecosystem for Big Neuro Data" Princeton University, Princeton, NJ, USA, 2018.
- [50]
J. T. Vogelstein. "Multiscale Graph Correlation: A Knowledge Representation System for Discovering Latent Geometric Structure" DARPA SIMPLEX PI Review Meeting, New York City, NY, USA, 2018.
- [49]
E. W. Bridgeford. "A High-Throughput Pipeline Identifies Robust Connectomes but Troublesome Variability" Organization of Human Brain Mapping, Suntec, Singapore, 2018.
- [48]
E. Perlman. "NeuroData: Embracing Open Source for Big Data Neuroscience" NSF NeuroNex Workshop on Super 3DEM, Austin, TX, USA, 2018.
- [47]
J. T. Vogelstein and V. Chandrashekhar. "NeuroNex + Stanford" NeuroNex-Stanford, Stanford, CA, USA, 2018.
- [46]
G. Kiar. "Connectome Coding: what is it, how do we do it, and why do we care?" Data science in Neuroscience Symposium, Suntec, Singapore, 2018.
- [45]
J. T. Vogelstein. "Lifelong Learning Forests" Darpa L2M PI Meeting, Arlington, VA, USA, 2018.
- [44]
J. T. Vogelstein. "Discovering Relationships and their Geometry Across Disparate Data Modalities" Yale University, New Haven, CT, USA, 2018.
- [43]
J. T. Vogelstein. "Connectome Coding" Schmidt Sciences, 2017.
- [42]
J. T. Vogelstein. "Discovering Relationships and their Geometry Across Disparate Data Modalities" Stanford University, Stanford, CA, US, 2017.
- [41]
D. Mhembere. "knor: a NUMA-Optimized In-Memory, Distributed and Semi-External-Memory k-means library" HPDC, Washington DC, USA, 2017.
- [40]
G. Kiar. "Science in the Cloud (SIC): A use-case in MRI Connectomics" Open Science Special Interest Group, Oxford University, Oxford, England, 2017.
- [39]
Y. Lee. "Network Dependence Testing via Diffusion Maps and Distance-Based Correlations" Joint Statistical Meetings, Baltimore, MD, USA, 2017.
- [38]
T. M. Tomita. "ROFLMAO: Robust Oblique Forests with Linear Matrix Operations" SIAM International Conference on Data Mining, Houston, TX, USA, 2017. [DOI]
- [37]
J. T. Vogelstein. "NeuroData: Enabling Terascale Neuroscience for Everyone" 3rd Annual BRAIN Iniative Investigators Meeting, Bethesda, MD, USA, 2016.
- [36]
C. Shen. "Multiscale Generalized Correlation" Joint Statistical Meeting, Chicago, IL, USA, 2016.
- [35]
J. T. Vogelstein. "NeuroData: Enabling Terascale Neuroscience for Everyone" Keystone Symposia: State of the Brain, Alpbach, Austria, 2016.
- [34]
C. Shen. "Local Distance Correlation for Testing Independence" Temple University, Philadelphia, PA, USA, 2015.
- [33]
J. T. Vogelstein. "Law of Large Graphs" DARPA Graphs, Columbia University, New York City, NY, USA, 2015.
- [32]
J. T. Vogelstein. "Research Computing Support for Neuroscience and Other Life Sciences" CASC, Aachen, Germany, 2015.
- [31]
J. T. Vogelstein. "From RAGs to Riches: Utilizing Richly Attributed Graphs to Reason from Heterogeneous Data" SIMPLEX Kickoff, New York City, NY, USA, 2015.
- [30]
J. T. Vogelstein. "From RAGs to Riches: Utilizing Richly Attributed Graphs to Reason from Heterogeneous Data: Part 1" DARPA SIMPLEX PI Meeting, New York City, NY, USA, 2015.
- [29]
J. T. Vogelstein. "From RAGs to Riches: Utilizing Richly Attributed Graphs to Reason from Heterogeneous Data: Part 2" DARPA SIMPLEX PI Meeting, New York City, NY, USA, 2015.
- [28]
J. T. Vogelstein. "Opportunities and Challenges in Big Data Neuroscience" DoE, 2015.
- [27]
J. T. Vogelstein and L. Paninski. "Spike inference from calcium imaging using sequential Monte Carlo methods" AMSI Program on Sequential Monte Carlo, 2015.
- [26]
J. T. Vogelstein. "big time (series data in neuroscience)" figshare, 2015.
- [25]
J. T. Vogelstein. "Top Challenges of Big Data Neuroscience" BRAIN Initiative Workshop, Bethesda, MD, USA, 2014.
- [24]
J. T. Vogelstein. "Big Statistics for Brain Sciences" Baylor College of Medicine, Department of Neuroscience, Houston, TX, USA, 2014.
- [23]
J. T. Vogelstein. "Beyond Little Neuroscience" Beyond Optogenetics workshop at Cosyne, Salt Lake City, UT, USA, 2013.
- [22]
J. T. Vogelstein. "Statistical Inference on Graphs" University of Michigan, Ann Arbor, Michigan, 2013.
- [21]
J. T. Vogelstein. "Statistical Inference on Graphs" Scientific Computing Institute, University of Utah, Salt Lake City, UT, USA, 2013.
- [20]
J. T. Vogelstein. "Open Problems in Neuropsychiatry" Data Seminar, Duke University, Durham, NC, USA, 2013.
- [19]
J. T. Vogelstein. "Statistical Models and Inference for big Brain-Graphs" NIPS Workshop on Acquiring and analyzing the activity of large neural ensembles, Lake Tahoe, NV, USA, 2013.
- [18]
J. T. Vogelstein. "BIG NEURO" Theory and Neurobiology, Duke University, Durham, NC, USA, 2012.
- [17]
J. T. Vogelstein. "Open Connectome Project" Academic Medical Center, Amsterdam, Netherlands, 2012.
- [16]
J. T. Vogelstein. "Are mental properties supervenient on brain properties" None, 2011.
- [15]
J. T. Vogelstein. "What can Translational neuroimaging Research do for Clinical Practice" Child Mind Institute, New York City, NY, USA, 2011.
- [14]
J. T. Vogelstein. "Statistical Connectomics" Harvard University Connectomics Labs, Cambridge, MA, USA, 2011.
- [13]
J. T. Vogelstein. "Once we get connectomes, what the \%\#* are we going to do with them?" Krasnow Institute for Advanced Study at George Mason Univeristy, Fairfax, VA, USA, 2011.
- [12]
J. T. Vogelstein. "Consistent Connectome Classification" Math/Bio Seminar, Duke University, Durham, NC, USA, 2011.
- [11]
J. T. Vogelstein. "Connectome Classification: Statistical Graph Theoretic Methods for Analysis of MR-Connectome Data" Organization for Human Brain Mapping, Quebec City, Canada, 2011.
- [10]
J. T. Vogelstein. "Consistent Graph Classification" Guest Lecture in Deisseroth Lab, Stanford University, Stanford, CA, USA, 2011.
- [9]
J. T. Vogelstein. "Neurocognitive Graph Theory" National Security Agency, 2009.
- [8]
J. T. Vogelstein. "OOPSI: A Family of Optimal OPtical Spike Inference Algorithms for Inferring Neural Connectivity from Population Calcium Imaging" Dissertation Defense, Johns Hopkins University, Baltimore, MD, USA, 2009.
- [7]
J. T. Vogelstein. "Sequential Monte Carlo in Neuroscience" SAMSI Program on Sequential Monte Carlo, Tracking Working Group, 2009.
- [6]
J. T. Vogelstein. "Towards Inference and Analaysis of Neural Circuits Inferred from Population Calcium Imaging" Guest Lecture in Schnitzer Lab, Stanford University, Stanford, CA, USA, 2009.
- [5]
J. T. Vogelstein. "Towards Inferring Neural Circuits from Calcium Imaging" Guest Lecture in Yuste Lab, Columbia University, New York City, NY, USA, 2009.
- [4]
J. T. Vogelstein. "Inferring Spike Trains Given Calcium-Sensitive Fluorescence Observations" Statistical Analysis of Neural Data, Pittsburgh, PA, USA, 2008.
- [3]
J. T. Vogelstein. "Inferring spike trains from Calcium Imaging" Redwood Center for Theoretical Neuroscience, University of California, Berkeley, CA, USA, 2008.
- [2]
J. T. Vogelstein. "Inferring spike trains from Calcium Imaging" Cambridge University, Gatsby Unit, and University College London, Cambridge, England, 2008.
- [1]
J. T. Vogelstein. "Model based optimal inference of spike times and calcium dynamics givern noisy and intermittent calcium-fluorescence observations" Neurotheory Center of Columbia University, New York City, NY, USA, 2007.
Abstracts/Poster Presentations
- [70]
L. A. De Silva and J. T. Vogelstein. "Kernel density networks" From Neuroscience to Artificially Intelligent Systems (NAISys), Cold Spring Harbor Laboratory, NY, USA, 2022. [URL]
- [69]
J. Dey, W. LeVine, L. A. De Silva, A. Geisa, and J. T. Vogelstein. "Out-of-distribution Detection Using Kernel Density Polytopes" From Neuroscience to Artificially Intelligent Systems (NAISys), Cold Spring Harbor Laboratory, NY, USA, 2022. [URL]
- [68]
J. J. How, G. Schuhknecht, M. B. Ahrens, F. Engert, and J. T. Vogelstein. "Transfer learning in larval zebrafish (Danio rerio)" From Neuroscience to Artificially Intelligent Systems (NAISys), Cold Spring Harbor Laboratory, NY, USA, 2022. [URL]
- [67]
B. D. Pedigo, M. Powell, E. W. Bridgeford, M. Winding, C. E. Priebe, and J. T. Vogelstein. "Generative network modeling reveals a first quantitative definition of bilateral symmetry exhibited by a whole insect brain connectome" From Neuroscience to Artificially Intelligent Systems (NAISys), Cold Spring Harbor Laboratory, NY, USA, 2022. [URL]
- [66]
J. M. Shin, L. Isik, and J. T. Vogelstein. "Measure of human-likelihood in tree-based ensemble model and artificial neural networks" From Neuroscience to Artificially Intelligent Systems (NAISys), Cold Spring Harbor Laboratory, NY, USA, 2022. [URL]
- [65]
H. Xu and J. T. Vogelstein. "Simplest streaming trees" From Neuroscience to Artificially Intelligent Systems (NAISys), Cold Spring Harbor Laboratory, NY, USA, 2022.
- [64]
E. W. Bridgeford, M. Powell, A. Alyakin, B. Caffo, and J. T. Vogelstein. "Batch Effects are Causal Effects: Applications in Human Functional Connectomes" Neuromatch 3, 2020.
- [63]
J. Chung, J. Dey, G. Kiar, C. E. Priebe, and J. T. Vogelstein. "Human Structural Connectomes are Heritable" Neuromatch 3, 2020.
- [62]
V. Gopalakrishnan, J. Chung, E. Bridgeford, J. Arroyo, B. D. Pedigo, C. E. Priebe, and J. T. Vogelstein. "Statistical Methods for Multiscale Comparative Connectomics" Neuromatch 3, 2020.
- [61]
B. D. Pedigo, M. Winding, T. Orujlu, M. Zlatic, Cardona,Albert, C. E. Priebe, and J. T. Vogelstein. "A quantitative comparison of a complete connectome to artificial intelligence architectures" NAIsys, Cold Spring Harbor, NY, USA, 2020.
- [60]
B. D. Pedigo, M. Winding, A. Saad-Eldin, T. Liu, A. Cardona, M. Zlatic, C. E. Priebe, and J. T. Vogelstein. "Statistical tools for nanoscale connectomics: clustering neurons in Drosophila larva brain and other applications" Neuromatch 3, 2020.
- [59]
R. Perry, J. Zorn, S. Czajko, D. S. Margulies, and J. T. Vogelstein. "Permutation-corrected independence testing for high-dimensional fMRI data" Neuromatch 3, 2020.
- [58]
A. Saad-Eldin, B. D. Pedigo, Y. Park, C. E. Priebe, and J. T. Vogelstein. "NeuroGraphMatch" Neuromatch 3, 2020.
- [57]
J. T. Vogelstein, H. Helm, B. D. Pedigo, R. Mehta, C. E. Priebe, and C. White. "A Biological Implementation of Lifelong Learning in the Pursuit of Artificial General Intelligence" NAIsys, Cold Spring Harbor, NY, USA, 2020.
- [56]
J. Cho, A. Korchmaros, J. T. Vogelstein, M. P. Milham, and T. Xu. "Developing a gradient flow framework to guide the optimization of reliability for the study of individual differences" OHBM and Resting State, Fairmont, Dallas, TX, USA, 2020.
- [55]
J. Cho, A. Korchmaros, J. T. Vogelstein, M. P. Milham, and T. Xu. "Impact of Concatenating fMRI Data on reliability for Functional Connectomics" OHBM and Resting State, Fairmont, Dallas, TX, USA, 2020.
- [54]
J. Hecheng, J. S. Ramirez, J. T. Vogelstein, M. P. Milham, and T. Xu. "Assessing functional connectivity beyond Pearson's correlation" Fairmont, Dallas, TX, USA, 2020.
- [53]
X. Li, J. Cho, M. P. Milham, and T. Xu. "Improving brain-behavior prediction using individual-specific components from connectivity-based shared response model" Resting State, Fairmont, Dallas, TX, USA, 2020.
- [52]
E. Bridgeford and J. T. Vogelstein. "Optimal Experimental Design for Big Data: Applications in Brain Imaging" OHBM, 2020.
- [51]
J. Cho, A. Korchmaros, J. T. Vogelstein, M. P. Milham, and T. Xu. "Impact of Concatenating fMRI Data on reliability for Functional Connectomics" OHBM and Resting State, Fairmont, Dallas, TX, USA, 2020.
- [50]
J. Cho, A. Korchmaros, J. T. Vogelstein, M. P. Milham, and T. Xu. "Developing a gradient flow framework to guide the optimization of reliability for the study of individual differences" OHBM and Resting State, Fairmont, Dallas, TX, USA, 2020.
- [49]
R. Perry and J. T. Vogelstein. "Identifying Differences Between Expert and Novice Meditator Brain Scans via Multiview Embedding" OHBM, 2020.
- [48]
B. Falk and J. T. Vogelstein. "NeuroData's Open Data Cloud Ecosystem" Harvard University, Cambridge, MA, USA, 2019. [URL]
- [47]
J. Chung, B. D. Pedigo, C. E. Priebe, and J. T. Vogelstein. "Clustering Multi-Modal Connectomes" OHBM, Rome Italy, 2019. [URL]
- [46]
J. Chung, B. D. Pedigo, C. E. Priebe, and J. T. Vogelstein. "Human Structural Connectomes are Heritable" OHBM, Rome Italy, 2019. [URL]
- [45]
J. Browne, D. Mhembere, T. M. Tomita, J. T. Vogelstein, and R. Burns. "Forest Packing: Fast Parallel Decision Forests" SIAM International Conference on Data Mining, Calgary, Alberta, Canada, 2019. [URL]
- [44]
B. D. Pedigo, J. Chung, E. W. Bridgeford, B. Varjavand, C. E. Priebe, and J. T. Vogelstein. "GraSPy: an Open Source Python Package for Statistical Connectomics" Max Planck /HHMI Connectomics Meeting Berlin, Germany, 2019. [URL]
- [43]
A. Baden, E. Perlman, F. Collman, S. Smith, J. T. Vogelstein, and R. Burns. "Processing and Analyzing Terascale Conjugate Array Tomography Data" Berlin, Germany, 2017. [URL]
- [42]
P. Perlman and E. Eric. "NEURODATA: ENABLING BIG DATA NEUROSCIENCE" Kavli, Baltimore, MD, USA, 2017. [URL]
- [41]
S. J. Smith, R. Burns, M. Chevillet, E. Lein, G. Sapiro, W. Seeley, J. Trimmer, J. T. Vogelstein, and R. Weinberg. "The Open Synaptome Project: Toward a Microscopy-Based Platform for Single-synapse Analysis of Diverse Populations of CNS Synapses" Society for Neuroscience, Chicago, IL, USA, 2015. [URL]
- [40]
V. Vogelstein and J. T. "Open Connectome Project NeuroData: Enabling Data-Driven Neuroscience at Scale" Society for Neuroscience, Chicago, IL, USA, 2015. [URL]
- [39]
S. Chen, J. T. Vogelstein, S. Lee, M. Lindquist, and B. Caffo. "High Dimensional State Space Model with L-1 and L-2 Penalties" ENAR 2015, Miami, FL, USA, 2015. [URL]
- [38]
S. Chen, K. Liu, Y. Yuguang, L. Seonjoo, M. Lindquist, B. Caffo, and J. T. Vogelstein. "A Sparse High Dimensional State-Space Model with an Application to Neuroimaging Data" Figshare, 2015. [URL]
- [37]
E. L. Deyer, H. L. Fernandes, W. G. Roncal, D. Gursoy, J. T. Vogelstein, X. Xiao, C. Jacobsen, K. P. Kording, and N. Kasthuri. "X-Brain: Quantifying Mesoscale Neuroanatomy Using X-ray Microtomography" Figshare, 2015. [URL]
- [36]
S. Wang, Z. Yang, X. Zuo, M. Milham, C. Craddock, C. E. Priebe, and J. T. Vogelstein. "Optimal Design for Discovery Science: Applications in Neuroimaging" Figshare, 2015. [URL]
- [35]
S. Sikka, S. A. Cheung, B. A. Khanuja, R. A. Ghosh, S. A. Yan, C. A. Li, Q. A. Vogelstein, J. A. Burns, R. A. Colcombe, S. A. Craddock, C. A. Mennes, M. A. Kelly, C. A. Dimartino, A. A. Castellanos, F. A. Milham, and M. Michael. "Towards automated analysis of connectomes: The configurable pipeline for the analysis of connectomes (c-pac)" 5th INCF Congress of Neuroinformatics, Munich, Germany, 2014. [URL]
- [34]
J. T. Vogelstein and C. E. Priebe. "Nonparametric Two-Sample Testing on Graph-Valued Data." Duke Workshop on Sensing and Analysis of HighDimensional Data, Durham, NC, USA, 2013.
- [33]
D. Koutra, Y. Gong, S. Ryman, R. Jung, J. T. Vogelstein, and C. Faloutsos. "Are All Brains Wired Equally?" Proceedings of the 19th Annual Meeting of the Organization for Human Brain Mapping (OHBM), Seattle, WA, USA, (4.2)1:3, 2013. [URL]
- [32]
M. Mhembere, D. A. Burns, R. A. Vogelstein, J. T. A. Vogelstein, R. J. A. Sussman, D. A. Priebe, C. A. Jung, R. Rex, A. Ryman, and S. Sephira. "Multivariate Invariants from Massive Brain-Graphs" OHBM, Seattle, WA, USA, 2013. [URL]
- [31]
Y. Qin, D. Mhembere, S. Ryman, R. Jung, R. J. Vogelstein, R. Burns, J. Vogelstein, and C. Priebe. "Robust Clustering of Adjacency Spectral Embeddings of Brain Graph Data via Lq-Likelihood" OHBM, Seattle, WA, USA, 2013. [URL]
- [30]
G. Roncal, W. A. Kleissas, D. M. A. Burck, J. M. A. Manavalan, P. A. Vogelstein, J. T. A. Perlman, E. A. Burns, R. A. Vogelstein, and R. Jacob. "Towards a Fully Automatic Pipeline for Connectome Estimation from High-Resolution EM Data" OHBM, Seattle, WA, USA, 2013. [URL]
- [29]
D. L. Sussman, D. Mhembere, S. Ryman, R. Jung, R. J. Vogelstein, R. Burns, J. T. Vogelstein, and C. E. Priebe. "Massive Diffusion MRI Graph Structure Preserves Spatial Information" OHBM, Seattle, WA, USA, 2013. [URL]
- [28]
R. D, A. A. Vogelstein, J. A. Caffo, B. A. Pekar, J. J. A. H. I, and S. Sair. "Reproducible differentiation of individual of individual subjects with minimal acquisition time via resting state fMRI" Proc ISMRM, Salt Lake City, UT, USA, 2013. [URL]
- [27]
S. Sismanis, N. A. Sussman, D. L. A. Vogelstein, J. T. A. Gray, W. A. Vogelstein, R. J. A. Perlman, E. A. Mhembere, D. A. Ryman, S. A. Jung, R. A. Burns, R. A. Priebe, C. E. A. Pitsianis, N. A. Sun, and X. X.. "Feature Clustering from a Brain Graph for Voxel-to-Region Classification" 5th Panhellic Conference on Biomedical Technology, Athens, Greece, 2013. [URL]
- [26]
P. Pnevmatikakis, E. A. A. Machado, T. A. Grosenick, L. A. Poole, B. A. Vogelstein, and J. T. A. P. Liam. "Rank-penalized nonnegative spatiotemporal deconvolution and demixing of calcium inaging data" COSYNE, Salt Lake City, UT, USA, 2013. [URL]
- [25]
J. T. Vogelstein and others. "Anomaly Screening and Clustering of Multi-OBject Movies via Multiscale Structure Learning" DARPA XDATA Colloquium, 2013.
- [24]
V. Vogelstein, J. A. Sikka, S. A. Cheung, B. A. Khanuja, R. A. Li, Q. A. Y. C, .. A. Priebe, C. A. Calhoun, V. A. Vogelstein, R. J. A. Milham, M. A. Burns, and R. R.. "BRAINSTORM towards clinically and scientifically useful neuroimaging analytics" Neuroinformatics, Munich, Germany, 2012. [URL]
- [23]
V. Vogelstein, J. T. A. Bock, D. A. Gray, W. A. Sussman, D. A. Burns, R. A. Kleissas, D. A. Marchette, D. A. Fishkind, D. E. A. Tang, M. A. Hager, G. A. Vogelstein, and R. J. A. P. C. E.. "Statistical Connectomics" Janelia Farm conference, Statistical Inference and Neuroscience, Loudoun County, VA, USA, 2012. [URL]
- [22]
G. Gray, W. R. A. Kleissas, D. M. A. Burck, J. M. A. Vogelstein, J. T. A. Perlman, E. A. Burlina, P. M. A. Burns, and R. A. V. R. Jacob. "Towards a Fully Automatic Pipeline for Connectome Estimation from High-Resolution EM Data" Cold Spring Harbor Laboratory, Neuronal Circuits, Cold Spring Harbor, NY, USA, 2012. [URL]
- [21]
W. R. Gray, J. A. Bogovic, J. T. Vogelstein, C. Ye, B. A. Landman, J. L. Prince, and R. J. Vogelstein. "Magnetic resonance connectome automated pipeline and repeatability analysis" Society for Neuroscience, Washington DC, USA, 2011. [URL]
- [20]
J. T. Vogelstein, D. E. Fishkind, D. L. Sussman, and C. E. Priebe. "Large graph classification: theory and statistical connectomics applications" IMA conference on Large Graphs, University of Minnesota, Minneapolis, MN, USA, 2011. [URL]
- [19]
J. T. Vogelstein, W. Gray, J. G. Martin, G. C. Coppersmith, M. Dredze, J. Bogovic, J. L. Prince, S. M. Resnick, C. E. Priebe, and R. J. Vogelstein. "Connectome Classification using statistical graph theory and machine learning" Society for Neuroscience, Washington DC, USA, 2011. [URL]
- [18]
J. T. Vogelstein, D. L. Sussman, M. Tang, D. E. Fishkind, and C. E. Priebe. "Dot product embedding in large (errorfully observed) graphs with applications in statistical connectomics" IMA conference on Large Graphs, University of Minnesota, Minneapolis, MN, USA, 2011.
- [17]
J. T. Vogelstein, E. Perlman, D. Bock, W. C. Lee, M. Chang, B. Kasthuri, M. Kazhdan, C. Reid, J. Lichtman, R. Burns, and R. J. Vogelstein. "Open Connectome Project: collectively reverse engineering the brain one synapse at a time" Neuroinformatics, Boston, MA, USA, 2011. [URL]
- [16]
J. T. Vogelstein, W. R. Gray, R. J. Vogelstein, J. Bogovic, S. Resnick, J. Prince, and C. E. Priebe. "Connectome Classification: Statistical Graph Theoretic Methods for Analysis of MR-Connectome Data" Organization for Human Brain Mapping, Quebec City, Canada, 2011. [URL]
- [15]
W. R. Gray, J. T. Vogelstein, J. Bogovic, A. Carass, J. L. Prince, B. Landman, D. Pham, L. Ferrucci, S. M. Resnick, C. E. Priebe, and R. J. Vogelstein. "Graph-Theoretical Methods for Statistical Inference on MR Connectome Data" DARPA Neural Engineering, Science and Technology Forum, San Diego, CA, USA, 2010. [URL]
- [14]
J. T. Vogelstein, C. E. Priebe, R. Burns, R. J. Vogelstein, and J. Lichtman. "Measuring and reconstructing the brain at the synaptic scale: towards a biofidelic human brain in silico" DARPA Neural Engineeering, Science and Technology Forum, San Diego, CA, USA, 2010. [URL]
- [13]
J. T. Vogelstein, J. Bogovic, A. Carass, W. Gray, J. Prince, B. Landman, D. Pham, L. Ferrucci, S. Resnick, C. E. Priebe, and R. Vogelstein. "Graph-Theoretical Methods for Statistical Inference on MR Connectome Data" Organization for Human Brain Mapping, Barcelona, Spain, 2010. [URL]
- [12]
J. T. Vogelstein, R. Vogelstein, and C. E. Priebe. "A Neurocognitive Graph-Theoretical Approach to Understanding the Relationship Between Minds and Brains" CSHL conference on Neural Circuits, Cold Shore Harbor, NY, USA, 2010. [URL]
- [11]
J. T. Vogelstein, Y. Mishchenki, A. Packer, T. Machado, R. Yuste, and L. Paninski. "Towards Confirming Neural Circuit Inference from Population Calcium Imaging" COSYNE, Salt Lake City, UT, USA, 2010. [URL]
- [10]
J. T. Vogelstein, Y. Mishchenki, A. Packer, T. Machado, R. Yuste, and L. Paninski. "Towards Inferring Neural Circuit Inference from Population Calcium Imaging" COSYNE, Salt Lake City, UT, USA, 2010. [URL]
- [9]
J. T. Vogelstein, Y. Mishchchenko, A. M. Packer, T. A. Machado, R. Yuste, and L. Paninski. "Towards Confirming Neural Circuits from Population Calcium Imaging" NIPS Workshop on Connectivity Inference in Neuroimaging, Whistler, BC, Canada, 2009. [URL]
- [8]
J. T. Vogelstein, Y. Mishchenki, A. Packer, T. Machado, R. Yuste, and L. Paninski. "Towards Inferring Neural Circuit Inference from Population Calcium Imaging" COSYNE, Salt Lake City, UT, USA, 2009. [URL]
- [7]
J. T. Vogelstein, B. Babadi, B. Watson, R. Yuste, and L. Paninski. "From Calcium Sensitive Fluorescence Movies to Spike Trains" Society for Neuroscience, Washington DC, USA, 2008. [URL]
- [6]
J. T. Vogelstein, B. Babadi, and L. Paninski. "Model-Based Optimal Inference of Spike-Times and Calcium Dynamics given Noisy and Intermittent Calcium-Fluorescence Imaging" COSYNE, Salt Lake City, UT, USA, 2008. [URL]
- [5]
J. T. Vogelstein and L. Paninski. "Inferring Spike Trains, Learning Tuning Curves, and Estimating Connectivity from Calcium Imaging" Integrative Approaches to Brain Complexity, 2008. [URL]
- [4]
J. T. Vogelstein, B. Jedynak, K. Zhang, and L. Paninski. "Inferring Spike Trains, Neural Filters, and Network Circuits from in vivo Calcium Imaging" Society for Neuroscience, San Diego, CA, USA, 2007. [URL]
- [3]
J. T. Vogelstein, K. Zhang, B. Jedynak, and L. Paninski. "Maximum Likelihood Inference of Neural Dynamics under Noisy and Intermittent Observations using Sequential Monnte Carlo EM Algorithms" COSYNE, Salt Lake City, UT, USA, 2007. [URL]
- [2]
J. T. Vogelstein and K. Zhang. "A novel theory for simultaneous representation of multiple dynamic states in hippocampus" Society for Neuroscience, San Diego, CA, USA, 2004.
- [1]
J. T. Vogelstein, L. Snyder, M. Warchol, and D. Angelaki. "Up-down asymmetry in memory guided saccadic eye movements are independent of head orientation in space" Society for Neuroscience, Orlando, FL, USA, 2002.
Educational Activities
Teaching Experience - Ongoing Courses
- Fall '23 Philosophy of Life - A Data Science Perspective EN.580.419, Course Director, JHU, enrollment 10.
- Spring '22 NeuroData Design II EN.580.438/638, Course Director, JHU, enrollment 20. [URL]
- Fall '21 NeuroData Design I EN.580.437/697, Course Director, JHU, enrollment 27. [URL]
- Spring '21 NeuroData Design II EN.580.438/638, Course Director, JHU, enrollment 30. [URL]
- Fall '20 NeuroData Design I EN.580.237/437/697, Course Director, JHU, enrollment 38. [URL]
- Spring '20 NeuroData Design II EN.580.438/638, Course Director, JHU, enrollment 32. [URL]
- Fall '19 NeuroData Design I EN.580.237/437/637, Course Director, JHU, enrollment 46. [URL]
- Spring '19 NeuroData Design II EN.580.438/638, Course Director, JHU, enrollment 18. [URL]
- Fall '18 NeuroData Design I EN.580.237/437/637, Course Director, JHU, enrollment 22. [URL]
- Spring '17 NeuroData Design II EN.580.238/438/638, Course Director, JHU, enrollment 14. [URL]
- Winter '17 BME Research Intersession EN.580.574, Course Director, JHU, enrollment 6. [URL]
- Fall '17 NeuroData Design I EN.580.247/437/637, Course Director, JHU, enrollment 15. [URL]
- Spring '16 The Art of Data Science EN.580.468, Course Director, JHU, enrollment 24. [URL]
- Fall '16 NeuroData Design I EN.580.437, Course Director, JHU, enrollment 16. [URL]
- Spring '15 Statistical Connectomics EN.580.694, Course Director, JHU, enrollment 26. [URL]
Teaching Experience - One-Time
- Spring '19 Systems Bioengineering II EN.580.422, Guest Lecturer, JHU, 2 Lectures.
- Spring '19 Computational Neuroscience AS.080.321, Guest Lecturer, JHU, 2 Lectures.
- Spring '18 Systems Bioengineering II EN.580.422, Guest Lecturer, JHU, 2 Lectures.
- Spring '18 Computational Neuroscience AS.080.321, Guest Lecturer, JHU, 2 Lectures.
- Spring '17 Systems Bioengineering II EN.580.422, Guest Lecturer, JHU, 2 Lectures.
- Spring '16 Systems Bioengineering II EN.580.422, Guest Lecturer, JHU, 2 Lectures.
- Winter '16 Introduction to Connectomics EN.600.221, Guest Lecturer, JHU, 1 Lecture.
- Fall '16 BME Modeling and Design EN.580.111, Guest Lecturer, JHU, 1 Lecture.
- Fall '15 Introduction to Computational Medicine Course Co-Director, JHU.
Educational Workshops
- Summer '19 DiPy Workshop 1 day lecture on statistical connectomics, Bloomington, Indiana [URL]
- Fall '18 Society for Neuroscience Annual Meeting 1 day lecture on statistical connectomics, Educational Workshop, San Diego, CA [URL]
- Fall '17 Society for Neuroscience Annual Meeting 1 day lecture on statistical connectomics, Educational Workshop, San Diego, CA [URL]
- Summer '16 CRCNS Course on Mining and Modeling of Neuroscience Data 2 day lecture on statistical connectomics, Redwood Center for Theoretical Neuroscience, University of California, Berkeley [URL]
Mentorship
Research Track Faculty Mentorship
- 07/19 – 08/20 Ronak Mehta MSE Research Assistant BME, JHU
Finalizing three manuscripts on (1) uncertainty forests, (2) time-series dependence quantification, and (3) lifelong learning forests
- 03/19 – 05/20 Anton Alyakin BSE Assistant Research Engineer BME, JHU
Worked on various problems in statistical graph inference
- 02/19 – 12/19 Hayden Helm MSE Assistant Research Faculty BME, JHU
Lead research efforts developing theory and methods for lifelong learning
- 08/16 – 08/18 Eric Perlman Ph.D. Assistant Research Faculty BME, JHU
ead Scientist in developing storage, transfer, and visualization solutions for large data in our cloud infrastructure
- 03/16 – 06/20 Jesse Patsolic MA Assistant Research Faculty BME, JHU
Lead developer converting our extensions to decision forests to be merged into sklearn
Staff Research Scientists
- 10/23 – Itsuki Ogihara BME Research Assistant MS, JHU
- 09/20 – 04/23 Jong Shin MSE Software Engineer BME, JHU
Currently investigating the effect of inductive bias innately coinciding with various machine learning models
- 03/20 – 08/22 Ali Geisa MS Research Assistant BME, JHU
Researching progressive and lifelong learning theory
- 06/19 – 08/20 Devin Crowley BS Research Assistant BME, JHU
Lead developer of our scalable Python implementation of LDDMM
- 06/18 – 12/19 Benjamin Falk Ph.D. Research Engineer BME, JHU
Lead software engineer, oversees all development projects, solely responsible for all cloud infrastructure
Postdoctoral Fellows
- 09/22 – 12/22 Adam Li Ph.D. Postdoctoral Fellow BME, JHU
- 11/20 – Javier Josue How Ph.D. Postdoctoral Fellow Neurosciences, UCSD
Javier studies how larval zebrafish learn how to perform a task under one situation, and use this knowledge to learn another task more quickly. He hopes to use this understanding of biological transfer learning to improve machine learning, which tends to be unable to complete this feat.
- 07/19 – 08/21 Austin Grave Ph.D. Post-doctoral Fellow Kavli NDI, JHU
Co-Advised by Prof. Richard Huganir, Department of Neuroscience. Working on understanding whole brain synaptic plasticity using genetic engineering and light microscopy imaging
- 07/19 – 08/21 Celine Drieu Ph.D. Post-doctoral Fellow Kavli NDI, JHU
Co-Advised by Assitant Prof. Kuchibhotla, Department of Psychological and Brain Sciences. Working on understanding learning and memory using two-photon calcium imaging
- 08/18 – 08/20 Jesús Arroyo Ph.D. Post-doctoral Fellow CIS, JHU
Worked on graph matching and joint graph embedding
- 07/18 – 07/20 Audrey Branch Ph.D. Post-doctoral Fellow Kavli NDI, JHU
Co-Advised by Prof Michela Gallagher, extending brain clearing experimental technology from mice to rats. Currently with a manuscript on biorxiv
- 09/16 – 08/18 Cencheng Shen Ph.D. Post-doctoral Fellow CIS, JHU
Developed Multiscale Graph Correlation, which is currently the premiere hypothesis testing framework, and about to be integrated into SciPy, by far the world's leading scientific computing package. Currently an Assistent Professor in Department of Statistics at University of Delaware, and still an actice collaborator and grantee
- 06/16 – 07/17 Guilherme Franca Ph.D. Post-doctoral Fellow CIS, JHU
Worked on non-parametric clustering, with an article about to be accepted in PAMI, the leading machine learning journal. Currently a postdoc for Rene Vidal
- 05/16 – 06/17 Leo Duan Ph.D. Post-doctoral Fellow CIS, JHU
Went on to do a second postdoc with Leo Dunson (who I did my second postdoc with). Currently an Assistant Professor at University of Florida
- 08/14 – 05/22 Tyler Tomita MSE Postdoctoral Fellow BME, JHU
Developed Sparse Projection Oblique Randomer Forest in his dissertation, currently the best performing machine learning algorithm on a standard suite of over 100 benchmark problems. Currenly a postdoc with Assistant Prof. Chris Honey of Psychology and Brain Sciences
Ph.D. Students
- 09/24 - Rodrigo Guerra MS PhD Student BME, The City College of New York
Psychedelics - all
- 7/2024 - Cecelia Shuai Bachelor PhD student Neuroscience, Johns Hopkins
I’m interested in lifelong learning and am now working on prospective learning in the simulated ecological environment. I also model cool animal learning data collected in my joint-mentor’s lab
- 8/23 - 12/23 Skyler Thomas BS, BSA PhD Student (Rotation) BME, JHU
Skyler is a rotation student in the lab. He is interested in applied mathematics and machine learning. He is currently working on prospective learning theory. He is currently a Ph.D. student in BME at JHU.
- 05/23 – Yuxin Bai MSE PhD Student BME, JHU
- 05/22 – 05/23 Jeremy Welland Ph.D. PhD Student (Rotation) BME, JHU
- 02/22 – Alice Qingyang Wang Bsc PhD candidate Neuroscience, JHU
- 01/22 – 01/23 Noga Mudrik Ph.D. PhD Student (Rotation) BME, JHU
- 08/21 – Ashwin De Silva BS PhD Student BME, University of Moratuwa
Statistical Machine Learning
- 01/21 – Haoyin Xu MSE PhD Student BME, JHU
A Research Assistant who was also a Master's student in the NeuroData lab, maintainer of proglearn, working on streaming trees and forests
- 08/20 – 08/22 Kaleab A. Kinfu MSE PhD Student BME, JHU
Kaleab studied double descent phenomena in decision forests and deep learning methods and developed 'Partition and Decode' – a framework that formalizes an implicit internal representation of several modern machine learning methods. He is currently a Ph.D. student in CS at JHU.
- 05/20 – Tingshan Liu B.A. PhD Student Math Neuro, Smith College
Implementing and applying clustering algorithms to the connectomes of inset nervous systems.
- 08/19 – 12/23 Eric Bridgeford BSE PhD Student Department of Biostatistics, JHU
Dissertation will focus on statistics of human connectomes and mitigating batch effects. Already first author on several manuscripts under review, and spearheads collaboration with Prof Brian Caffo at Biostatistics
- 08/19 – 04/22 Mike Powell MSE PhD Candidate BME, Johns Hopkins University
Mike has studied drug-repurposing options for potential COVID-19 treatments, proposed methodological improvements and best practices for drug-repurposing studies, and developed a taxonomy for describing and quantifying feature importance in machine learning models.
- 07/19 – 06/24 Jayanta Dey MSE PhD Student BME, JHU
Currently working on lifelong learning that aims at training a machine learning model on multiple tasks and transferring knowledge among tasks
- 06/19 – 12/24 Sambit Panda MSE PhD Student BME, JHU
A Ph.D. student who was also a Master's student in the NeuroData lab. Currently, the maintainer of the `hyppo` package, and works on creating more powerful and efficient multivariate hypothesis tests.
- 05/19 –- 07/24 Jaewon Chung MSE PhD Student BME, JHU
Data science for macroscale connectomes. Co-creator and maintainer of `graspologic`, a Python package for network statistics.
- 01/19 – 01/24 Thomas Athey BS PhD Candidate BME, JHU
Tommy analyzes terabyte-scale full brain images from light microscopy with a focus on neuromorphology. His expertise is in statistics and computer vision.
- 08/18 – 05/23 Ben Pedigo BS PhD Candidate BME, JHU
Data science for nanoscale connectomes. Co-creator and maintainer of `graspologic`, a Python package for network statistics.
- 08/18 – 06/2022 Meghana Madyastha BSE PhD Co-advisee CS, JHU
Dissertation will focus on computational aspects of accelerationg learning and inference using decision forests
- 08/16 – 12/21 Vikram Chandrashekhar BSE PhD advisee BME, JHU
Dissertation has focused on extending LDDMM to whole cleared brain datasets, spearheads collaboration with Prof. Karl Deisseroth’s lab at Stanford, one of the world’s leading neuroscientists
Visiting Doctoral Student
- 03/19 – 09/19 Derek Pisner MSE PhD advisee JHU/UT, Austin
Worked on the ndmg pipeline, developing direct streamline normalization for structural connectome generation
M.S. Students
- 05/25 – Yilin (Clark) Xu BS MS Student BME, JHU
Dedicated on Treeple acceleration
- 05/25 - Yue (Zoe) Wan BS MS student AMS, JHU
- 4/25 – Beiya (Alexa) Xu MSE MS student BME, JHU
- 11/24 - Z. Qin MS MS student BME, JHU
- 9/24 – Siyu (Rice) Yu MSE MS student BME, JHU
- 6/23 – 8/24 Ziyan Li MSE MS student BME, JHU
- 05/20 – 12/21 Ali Saad-Eldin BSE MS advisee BME, JHU
Working on implementing and improving cominatorial optimization algorithms, specifically the Quadratic Assignment Problem
- 02/20 – 12/20 Will LeVine MS advisee BME, JHU
Exploring different sub-algorithms within progressive learning to alleviate harmful effects that resultfrom training on unhelpful data
- 01/20 – 08/22 Shreya Singh BS Graduate Researcher BME, JHU
brainlit' package, aws and azure data management
- 07/19 – 04/22 Ross Lawrence BSE MS advisee BME, JHU
Lead m2g developer, maintainer of neuroparc, MRI connectome repositories
- 06/19 – 12/20 Bijan Varjavand BSE MS advisee BME, JHU
Submitted manuscript to PAMI on advancing statistics on populaitons of networks
- 06/19 – 05/21 Vivek Gopalakrishnan MSE Combined BS/MSE Student BME, JHU
Vivek developed multiscale hypothesis tests for multi-subject connectomics datasets, and is currently a PhD student in Medical Engineering and Medical Physics at the Harvard-MIT Program in Health Sciences and Technology.
- 01/19 – 06/21 Ronan Perry MSE MSE/BS Student BME, JHU
Ronan studied random forest methods for structured data and improved prediction calibration, developed nonparametric hypothesis testing tools, and explored novel complexity measures of neural networks. He is currently a Fulbright Germany scholar with Bernhard Scholkopf.
- 10/18 – 04/22 Alex Loftus BSE MS advisee BME, JHU
graph stats book, 'graspologic' package, ndmg development
- 06/18 – 06/19 Drishti Mannan BSE MS advisee BME, JHU
Preparing manuscript introducing novel specification for large attributed networks
- 08/14 – 06/17 Greg Kiar BSE MSE advisee BME, JHU
Developer of m2g, the only existing "soup to nuts" pipeline for both functional and diffusion pipelines, co-first author of manuscript under review. Currently a PhD student at McGill University
Undergraduate Students
- 6/23 – 12/23 Anvii Mishra BS Undergraduate BME, JHU
- 10/22 –- Hope Ugwuoke BS Undergraduate BME, JHU
- 06/22 – 12/22 Audrey Herskovits BS Undergraduate (Visiting) BME, JHU
- 06/22 – 02/23 Sejal Srivastava BS Undergraduate BME, JHU
- 09/21 – 08/24 Kareef Ullah Undergraduate Researcher BME, JHU
Assisted with fixing issues in graspologic and hyppo
- 08/20 – 05/21 Alisha Kodibagkar Undergraduate Researcher BME, JHU
Assisting in the integration of brainlit packages with Azure services
- 05/20 – 06/2022 Diane Lee Undergraduate Researcher BME, JHU
Assisting in the maintenance of graspologic
- 06/21 – 08/21 Dominique Allen Undergraduate Researcher BME, JHU
Assisted Thomas Athey in his work with statistics and computer vision
- 06/19 – 12/19 Richard Guo Undergraduate Researcher BME, JHU
Developed uncertainty forests, an approach for estimated posterior class probabilities, conditional entropy, and mutual information for high-dimensional data common in brain science applications
- 06/15 – 08/16 Albert Lee BSE Undergraduate BME, JHU
Developed big data visualization tools
- 06/15 – 12/15 Ron Boger BSE Undergraduate Researcher BME, JHU
Worked at a computational medicine start-up in Silicon Valley, worked on high-dimensional low-sample size theory
- 05/15 – 05/16 Jordan Matelsky BSE BME, JHU
Currently a data scientist at APL, developed a number of simple WebApps in support of big data management
- 02/15 – 05/16 Ivan Kuznetsov BSE BME, JHU
Currently an MD, PhD Candidate at the UPenn, winner of Soros Fellowship, worked on analysis of data from Dr. Daniel Amen, developed matrix exploratory data analysis package.
Highschool Student
Thesis Committee Service (BME unless noted otherwise)
- 2019 Browne, James Computer Science JHU Ph.D. Student, Graduated 2019
- 2019 Mhembere, Disa Computer Science JHU Ph.D. Student, Graduated 2019
- 2018 Kutten, Kwame JHU Ph.D. Student, Graduated 2018
- 2018 Wang, Shangsi Applied Mathematics and Statistics JHU Ph.D. Student, Graduated 2018
- 2018 Tang, Runze Applied Mathematics and Statistics JHU Ph.D. Student, Graduated 2018
- 2018 Lee, Youjin Biostatistics JHU Ph.D. Student, Graduated 2018
- 2017 Zheng, D Computer Science JHU Ph.D. Student, Graduated 2017
- 2017 Binkiewicz, Norbert Statistics University of Wisconsin Ph.D. Student, Graduated 2017
- 2016 Gray-Roncal, Will Computer Science JHU Ph.D. Student, Graduated 2016
Service
Grant Review Service
- 2015 NSF Review Panel Review for NSF BIG DATA Program
University Service
- Winter '19 Track Organizer AI in Healthcare: From Bench to Bedside
Organizer for Breakout Topic Sessions on artificial intelligence
- 08/15 – 07/18 Co-Developer Computational Medicine Minor, JHU, Baltimore, MD, USA
http://icm.jhu.edu/academics/undergraduate-minor/
- 2015 – 2017 Co-Founder and Faculty Advisor MedHacks
http://medhacks.org/ Medhacks is one of the first and largest hackathons dedicated specifically to hacking on medical advances, started entirely by BME undergrads at JHU
- 08/14 – 08/18 Director of Undergraduate Studies Institute for Computational Medicine, JHU, Baltimore, MD, USA
http://icm.jhu.edu/academics/undergraduate-minor/
Department Service
- 2019 Member Search Committee, BME, Neuroengineering, 2019
- 2019 Member Search Committee, BME, Data Science, 2019
- 2018 Member Search Committee, BME, Neuroengineering, 2018
Service in Scientific Community
- 2023 – Member National Advisory Mental Health Council Workgroup on High-Dimensional Datasets
- 2019 – Mentor Black in AI
- 2017 – Scientific Advisory Board NSF NeuroNex
Enhanced resolution for 3DEM analysis of synapses across brain regions and taxa. Provided scientific, computational, and statistical guidance to a flagship NSF funded BRAIN Initiative program
- 2017 – Chair of Committee of Data Cores U19 Data Cores
The U19 program is NIH's flagship BRAIN Initative program, with five original awardees, each with a dedicated Data Core and designated PI. I was elected the chair of the committee of Data Core PIs
- 2017 Consultant for Nature Publishing Group The journal Nature
The journal Nature, flagship journal of Nature Publishing Group, decided to create a ``Code and Software Submission Checklist''. They consulted me on their first draft, and I helped re-write it.
- 2011 – Open Connectome Project
The co-founder of the ``Open Connectome Project'' (OCP), for several years, I was the only neuroscientist that could easily store, manage, and analyze very big datasets, spanning first tens of terabytes, and then hundreds. For that reason, I was an essential co-author on a number of big data papers. Specifically, though I sometimes contributed relatively little to the scientific ideas, I often was required to complete, visualize, and/or share the data. Perhaps more importantly, both funding agencies and journals began mandating that these large datasets be publicly shared, and OCP was literally the only option. This is despite often not having funding, nor being a co-author, on the manuscripts
- 2010 – AWS Open Neuro Data Registry
Our lab co-founded the https://registry.opendata.aws/open-neurodata/ Registry of Open Data on Amazon Web Serivces (AWS). The implication of this is that now, pending a few minor considerations, any neuroscientist that collects large image data can deposit it online for free. This means that neither they nor we must request funding to store the data. Our lab maintains this repository, but only by virtue of ensuring instructions for uploading, visualizing, and downloading are up to date, and acting as a gatekeeper to ensure only appropriate data are deposited there
Journal Service: Editorial Board
- 2019 – Associate Editor
Journal of the American Statistical Association
- 2018 – Editor
Neurons, Behavior, Data analysis, and Theory
- 2016 Guest Associate Editor
PLoS Computational Biology
Journal Service: Conference and Journal Reviewer
- Neural Information Processing Systems (NeurIPS)
- International Conference on Learning Representations (ICLR)
- International Conference on Machine Learning (ICML)
- Annals of Applied Statistics (AOAS)
- Bioinformatics
- International Conference on Learning Representations (ICLR)
- Network Science
- Current Opinion in Neurobiology
- Biophysical Journal
- IEEE International Conference on eScience
- IEEE International Conference on Acoustics, Speech, and Signal Processing (ICASSP)
- IEEE Global Conference on Signal and Information Processing (GlobalSIP)
- IEEE Signal Processing Letters
- IEEE Transactions on Signal Processing
- Frontiers in Brain Imaging Methods
- Journal of Machine Learning Research (JMLR)
- Journal of Neurophysiology
- Journal of the Royal Statistical Society B (JRSSB)
- Nature Communications
- Nature Methods
- Nature Reviews Neuroscience
- Neural Computation
- Neural Information Processing Systems (Neurips)
- NeuroImage
- Neuroinformatics
- PLoS One
- PLoS Computational Biology
Conferences and Hackathon Organizer
- Summer '20 Co-Chair SciPy mini-symposium: Biology and Bioinformatics
- Winter '19 Organizer Decision Forest Hackathon
- Summer '19 Organizer NeuroData Workshop
https://neurodata.devpost.com/ Hackashop to train brain scientists in machine learning for big data (50) participants from around the country
- March '19 Organizer Neuro Reproducibility Hackashop
https://brainx3.io/ Hackashop to train brain scientists in best practices in reproducible science, co-organized with two startups: Vathes, LLC and Gigantum (50 participants)
- Spring '18 Organizer NeuroData Hackathon
- Fall '17 Organizer NeuroData Mini-Hackathon
- Summer '17 Organizer NeuroStorm
https://brainx2.io/ Workshop to bring together thought leaders from academia, national labs, industry, and non-profits around the world to take next steps towards accelerating brain science discovery in the cloud (50 participants and 5 observers from funding institutions)
- 2016 Organizer Global Brain Workshop
http://brainx.io/ First ever international Brain Initiative workshop, bringing together leaders from around the world, covered by Nature and Science (75 participants)
- 2016 Co-Organizer Brains and Bits: Neuroscience Meets Machine Learning, NIPS Workshop
http://www.stat.ucla.edu/~akfletcher/brainsbits_overview.html
- Winter '15 Organizer Hack@NeuroData
http://hack.neurodata.io/
- 2015 Co-Organizer BigNeuro2015: Making Sense of Big Neural Data, NIPS Workshop
http://neurodata.io/bigneuro2015
- 2012 Co-Organizer Scaling up EM Connectomics Conference
https://openwiki.janelia.org/wiki/download/attachments/8687459/final+agenda+EM+Connectomics+100512.pdf The world's first connectomics workshop, now run annually alternating between Janelia Research and Max Plank locations (80 participants)
Awards and Recognition
Individual
- 2002 Dean’s List Washington University
Shared
- 2019 Kavli NDI Distinguished Postdoctoral Fellow Celine Drieu, PhD
- 2019 Kavli NDI Distinguished Postdoctoral Fellow Austin Graves, PhD
- 2019 Kavli NDI Distinguished Postdoctoral Fellow Audrey Branch, PhD
- 2019 Winner of Pistritto Fellowship. Vivek Gopalakrishnan
- 2017 Best Presentation Award HPDC Mhembere et al.
- 2017 Nonparametric Statistics of the American Statistical Association Student Paper Award Lee et al.
- 2014 F1000 Prime Recommended Vogelstein et al.
- 2013 Spotlight Neural Information Processing Systems (NIPS)
- 2011 Trainee Abstract Award Organization for Human Brain Mapping
- 2008 Spotlight Computational and Systems Neuroscience (CoSyNe)
Other Media
- [31] NPR "Why scientists just mapped every synapse in a fly brain" NPR [URL]
- [30] Science Friday "The First Fully Mapped Animal Brain Is The Larva Of A Fruit Fly" WNYC Studio [URL]
- [29] Hamblin, James "Why Some People Get Sicker Than Others: COVID-19 is proving to be a disease of the immune system." The Atlantic [URL]
- [28] Ladapo, Joseph A. "Too Much Caution Is Killing Covid Patients" The Wall Street Journal [URL]
- [27] Mandavilli, Apoorva "Coronavirus Can Set Off a 'Cytokine Storm.' These Drugs May Calm It" The New York Times [URL]
- [26] Mone, Amy and Mehl, Valerie "Researchers Urge Clinical Trial of Blood Pressure Drug to Prevent Lethal Complication of Covid-19" Johns Hopkins Medicine [URL]
- [25] Rosen, Meghan "Preventing 'Cytokine Storm' May Ease Severe COVID-19 Symptoms" Howard Hughes Medical Institute [URL]
- [24] Salzberg, Steven "Prazosin Might Be A Treatment for COVID-19. More Data Is Urgently Needed" Forbes [URL]
- [23] Johns Hopkins Medicine "BME Pioneers: Joshua Vogelstein" BME Pioneers [URL]
- [22] Johns Hopkins Medicine "Technology Connecting the Brain to the Human Experience" Tomorrows Discoveries [URL]
- [21] Perkel, Jeffrey M. "Web service makes big data available to neuroscientists" Nature [URL]
- [20] Emerging Technology from the arXiv "Three Grand Challenges for Brain Science That Can Be Solved in 10 Years" MIT Technology Review [URL]
- [19] Patel, Prachi "Johns Hopkins researchers want to use big data to chart the brain" Johns Hopkins University [URL]
- [18] Reardon, Sara "Worldwide brain-mapping project sparks excitement --- and concern" Nature [URL]
- [17] Underwood, Emily "International brain projects proposed" Science [URL]
- [16] {National Institues of Health} "International Brain Projects Considered" BRAIN initiative [URL]
- [15] {Office of the Spokesperson} "International Brain Initiative Launch and VIP Dialog: Towards an International Brain Station" US Department of State [URL]
- [14] {The Kavli Foundation} "International Brain Initiative" Kavli [URL]
- [13] Dale Keiger "The Open Connectome Project takes a close look at the brain" Johns Hopkins Magazine [URL]
- [12] Begley, Sharon "Fly brain 'atlas' opens door to linking human neurons to actions" Reuters [URL]
- [11] Gatlin, Latarsha "Johns Hopkins mathematician receives grant to support study of brain's circuitry" Johns Hopkins University [URL]
- [10] O'Leary, Timothy and Marder, Eve "Mapping Neural Activation onto Behavior in an Entire Animal" Science [URL]
- [9] Sanders, Laura "Ten thousand neurons linked to behaviors in fly" ScienceNews [URL]
- [8] Son, Donghee and Lee, Jongha "Research Highlights" Nature [URL]
- [7] Yandell, Kate "Linking Neurons to Behaviors" TheScientist [URL]
- [6] Yirka, Bob "Researchers create a reference atlas for neural circuits in fruit fly larvae" MedicalXpress [URL]
- [5] Begg, Colin B. and Pike, Malcolm C. "Comment on "The Predictive Capacity of Personal Genome Sequencing"" Science [URL]
- [4] Thomas, Ben "“Open Access to the Brain” – Podcast 1: Joshua Vogelstein" The Connectome Podcast [URL]
- [3] Topol, Eric J. "Comment on "The Predictive Capacity of Personal Genome Sequencing"" Science [URL]
- [2] Birch, Kristi "Mapping the Mind" Johns Hopkins Engineering [URL]
- [1] Vogelstein, Joshua T. "Q\&A: What is the Open Connectome Project?" BMC Nature [URL]
Professional/Social Media Presence
- @neuro_data Twitter account with a approximately 7,000 followers, over 250K impressions in December 2019, and approximately 100 new followers, and upwards of 100 new tweets, per month, and 25 link clicks per day. Follower demographics include <50% high school graduates, 46% female
- Bits and Brains Professional blog reguarding all things academic, neurological, and statistical, with approximately 30 blog posts, approximately one new post per month (9,000 page views, 3,200 unique users)
Most Popular Post: 10 Simple Rules to Write a Paper from Start to Finish - medium.com/@progl My Medium account where I post articles on both personal and professional topics
Translation / Technology Transfer Activities
Open Datasets
- 2019 – Templier et al. (2019) [URL]
The non-destructive collection of ultrathin sections onto silicon wafers for post-embedding staining and volumetric correlative light and electron microscopy using MagC. MagC allows the correlative visualization of neuroanatomical tracers within their ultrastructural volumetric electron microscopy context
0 citations, 119 unique visitors
- 2018 – Bloss et al. (2018) [URL]
Images of CA1 pyramidal neurons for analysis involving feature-selective firing as a result of dendritic integration of inputs from multiple brain regions. Show that single presynaptic axons form multiple, spatially clustered inputs onto the distal, but not proximal, dendrites of CA1 pyramidal neurons
20 citations, 530 unique visitors
- 2018 – Branch (2018) [URL]
Adult generated neurons in aging M. musculus imaged using array tomography, multi-spectral light microscopy, and electron microscopy
2 citations, 223 unique visitors
- 2017 – Allen Atlas [URL]
Anatomical reference atlases that illustrate the adult mouse brain in coronal and sagittal planes. They are the spatial framework for datasets such as in situ hybridization, cell projection maps, and in vitro cell characterization. http://atlas.brain-map.org/
142 citations, 1058 unique visitors
- 2017 – Hildebrand et al. (2017) [URL]
A multi-resolution serial-section electron microscopy data set containing the anterior quarter of a 5.5 days post fertilization larval zebrafish, including its complete brain acquired by Hildebrand and colleagues. Electron micrographs and reconstructions are available for view in CATMAID
70 citations, 1,014 unique visitors
- 2017 – Tobin et al. (2017) [URL]
Wiring variations that enable and constrain neural computation in a sensory microcircuit
28 citations, 43 unique visitors
- 2016 – Bloss et al. (2016) [URL]
Images of molecularly defined inhibitory interneurons and CA1 pyramidal cell dendrites collected using correlative light-electron microscopy and large-volume array tomography
41 citations, 701 unique visitors
- 2016 – Dyer et al. (2016) [URL]
Mesoscale (1 cubic micron resolution) resolution images generated with the use of synchrotron X-ray microtomography (microCT) from millimeter-scale volumes of mouse brain. X-ray tomography promises rapid quantification of large brain volumes
21 citations, 216 unique visitors
- 2016 – Lee et al. (2016) [URL]
Electron microscopy data collected at $4 \times 4 \times 40$ nm per voxel from the visual cortex in Mouse V1 used in a study of an excitatory network
132 citations, 725 unique visitors
- 2016 – Wanner et al. (2016 [URL]
Serial block face scanning EM (SBEM) and conductive sample embedding image stack from an olfactory bulb (OB) of a zebrafish larva at a voxel resolution of $9.25 \times 9.25 \times 25$ nm3
12 citations, 328 unique visitors
- 2015 – Amunts et al. (2015) [URL]
BigBrain is an ultrahigh-resolution three-dimensional model of a full human brain at 20 micrometer resolution, enabling an unprecedented look into the human brain at micro- and macro-scopic scale
262 citations, 1,041 unique visitors
- 2015 – Bhatla et al. (2015) [URL]
Nikhil Bhatla and Rita Droste in Bob Horvitz's Lab reconstruction of the anterior half of the C. elegans feeding organ, the pharynx. Volumes for three adult hermaphrodite worms include volumetric tracing of all neurons, selected cell types, I2 neuron synapses. 50 nm thick sections with an image resolution of 2 nm per pixel
16 citations, 467 unique visitors
- 2015 – Collman et al. (2015) [URL]
Mouse cortex collected using conjugate array tomography (AT), a volumetric imaging method that integrates immunofluorescence and EM imaging modalities in voxel-conjugate fashion
69 citations, 382 unique visitors
- 2015 – Deisseroth et al. (2015) [URL]
Twelve CLARITY mouse brains (5 wild type controls and 7 behaviorally challenged) were prepared by Li Ye, and imaged using CLARITY-Optimized Light-sheet Microscopy (COLM) (whole brain COLM imaging and data stitching performed by R. Tomer, in preparation)
5 citations, 208 unique visitors
- 2015 – Harris et al. (2015) [URL]
Three volumes of hippocampal CA1 neuropil in adult rat imaged by the laboratory of Kristen M Harris, PhD, at an XY resolution of ~2 nm on serial sections of ~50-60 nm thickness
9 citations, 463 unique visitors
- 2015 – Kasthuri et al. (2015) [URL]
Saturated reconstruction of a sub-volume of mouse neocortex collected using automated technologies in which all cellular objects (axons, dendrites, and glia) and many sub-cellular components are rendered and itemized in a database. Provides access to the complexity of the neocortex and enables further data-driven inquiries
323 citations, 1,299 unique visitors
- 2015 – Micheva et al. (2015) [URL]
Multi-channel array tomography data of the barrel cortex of an adult mouse (C57BL/6J)
57 citations, 190 unique visitors
- 2015 – Ohyama et al. (2015) [URL]
The side view of the approximately 7,000 neurons reconstructed so far, either in full or partially, of the approximately 12,000 neurons of the central nervous system of Drosophila larva. The 0111-8 data set was originally sectioned and imaged by Richard D. Fetter and his two tech assistants
136 citations, 299 unique visitors
- 2015 – Randlett et al. (2015) [URL]
Zebrafish brain atlas with surface mesh of different regions intended for the analysis of whole-brain activity mapping
124 citations, 498 unique visitors
- 2014 – Weiler (2014) [URL]
Images of whisker-associated barrel columns of mouse somatosensory cortex stained with antibodies against selected antigens (DAPI, YFP), and indirect immunofluorescence. Images collected by the lab of Stephen J Smith
6 citations, 123 unique visitors
- 2013 – Bumbarger et al. (2013) [URL]
Serial, thin section data generated by Dan Bumbarger in Ralf Sommer's lab in order to compare the pharyngeal connectomes of the pharyngeal nervous system between Caenorhabditis elegans and Pristionchus pacificus. In P. pacificus they found clearly homologous neurons for all of the 20 pharyngeal neurons in C. elegans, and massive rewiring of synaptic connectivity between the two species
67 citations, 22 unique visitors
- 2013 – Takemura et al. (2013) [URL]
The right part of the brain of a wild-type Oregon R female fly that was serially sectioned into 40-nm slices. A total of 1,769 sections, traversing the medulla and downstream neuropils, were imaged at a magnification of 35,000X
323 citations, 144 unique visitors
- 2011 – Bock et al. (2011) [URL]
Volume of mouse primary visual cortical data, spanning layers 1, 2/3, and upper layer 4 collected as electron microscope (EM) data and two-photon microscopy data collected by Davi Bock, Ph.D. and Wei-Chung Allen Lee, Ph.D.. Images have a resolution of 4x4x45 cubic nanometers
430 citations, 511 unique visitors
Open-Source Software: Active
- 2021 – scikit-tree [URL]
Scikit-tree is a package for modern tree-based algorithms for supervised and unsupervised learning problems. It extends the robust API of scikit-learn for tree algorithms that achieve strong performance in benchmark tasks.
38 stars, 7 forks
- 2020 – hyppo ((HYPothesis Testing in PythOn) [URL]
An open-source software package for multivariate hypothesis testing. It intends to be a comprehensive multivariate hypothesis testing package that runs on all major versions of operating systems. It also includes novel tests not found in other packages.
201 stars, 82 forks
- 2020 – brainlit [URL]
This repository is a container of methods that Neurodata usees to expose their open-source code while it is in the process of being merged with larger scientific libraries such as scipy, scikit-image, or scikit-learn.
23 stars, 17 forks
- 2019 – graspologic (Graph Statistics) [URL]
Co-developed with Microsoft Research: Utilities and algorithms designed for processing and analysis of graphs with specialized graph statistical algorithms
134 stars, 56 forks, 2,516 downloads/month
- 2019 – neuroparc [URL]
This repository contains a number of useful parcellations, templates, masks, and transforms to (and from) MNI152NLin6 space. The files are named according to the BIDs specification
26 stars, 4 forks
- 2018 – m2g (MR graph analysis) [URL]
A Python pipeline which uses diffusion MRI data from individuals to generate connectomes reliably and scalably
35 stars, 26 forks, 218 downloads/month, 7,900 docker pulls
Open-source Software: Contributed
- 2019 cloud-volume [URL]
Added support for additional file types
- 2019 – C-PAC [URL]
Added streamlined reproducible pipeline
- 2019 – scipy [URL]
Added mgc, a state of the art method for hypothesis testing we developed in the lab
- 2018 – 2019 neuroglancer [URL]
Added multispectral support to enable light microscopy data use
- 2018 – igraph [URL]
Added spectral clustering functionality
- 2017 – 2018 render [URL]
Added cloud support
- 2017 boss [URL]
Developed core functionality
Open-source Software: Archived
- 2020 – 2022 ProgLearn (Progressive Learning) [URL]
A Python package for exploring and using progressive learning algorithms
22 stars, 29 forks, 37 downloads/month
- 2019 – 2020 ARDENT (Affine and Regularized Deformative Numeric Transform) [URL]
A Python package for performing automated image registration using LDDMM
10 stars, 5 forks
- 2019 – 2021 reg (Image registration) [URL]
A Python package which performs non-linear affine and deformable image registration
6 stars, 4 forks, 61 downloads/month
- 2019 – 2019 https://neurodata.io/forests/ [URL]
SPORF is an improved random forest algorithm that achieves better accuracy and scaling than previous implementations on a standard suite of > 100 benchmark problems
54 stars, 35 forks, 73 downloads/month, 36 docker pulls
- 2019 – 2020 Uncertainty-Forest [URL]
A Python package containing estimation procedures for posterior distributions, conditional entropy, and mutual information between random variables X and Y
2 stars, 1 fork
- 2018 – 2021 LOL (Supervised dimensionality reduction) [URL]
Linear Optimal Low-rank (LOL) projection for improved classification performance in high-dimensional classification tasks
8 stars, 6 forks, 60 downloads/month
- 2018 – 2021 MGC (Non-parametric hypothesis testing) [URL]
Multiscale Graph Correlation (MGC) is a framework for universally consistent testing high-dimensional and non-Euclidean data
28 stars, 11 forks, 120 downloads/month, 266 docker pulls
- 2018 – 2019 ndcloud (NeuroData Cloud) [URL]
The deployment of tools which support the Open Connectome Project
- 2016 – 2019 Non-Parametric-Clustering [URL]
A program which uses non-parametric-clustering to minimize or maximize a given criterion function
3 stars, 2 forks
- 2017 – 2019 ndex [URL]
Python 3 command-line program to exchange (download/upload) image data with NeuroData's cloud deployment of APL's BOSS spatial database
3 stars, 0 forks, 89 downloads/month
- 2017 – 2019 knor (Clustering) [URL]
Python version of knor, a highly optimized and fast library for computing k-means in parallel with accelerations for Non-Uniform Memory Access (NUMA) architectures
1 stars, 3 forks, 115 downloads/month
- 2017 – 2019 SynapseAnalysis (Synapse Detection) [URL]
A framework to evaluate synaptic antibodies for array tomography applications
2 stars, 0 forks
- 2017 – 2018 MEDA (Matrix Exploratory Data Analysis) [URL]
A python package for matrix exploratory data analysis
0 stars, 3 forks, 56 downloads/month, 21 docker pulls
- 2017 – 2018 ndwebtools [URL]
ndwebtools (ndweb) is a Django application to provide a user-friendly interface for interacting with NeuroData resources and data
0 stars, 1 forks
- 2015 – 2018 ndviz [URL]
Web visualization and analysis tools for neuroimaging datasets, powered by Neuroglancer
8 stars, 4 forks, 48 docker pulls
- 2015 – 2016 DMG [URL]
An implementation of a distributed multigrid Poisson solver for image stitching, smoothing, and sharpenting
19 stars, 6 forks
- 2015 VESICLE (EM Synapse Detection) [URL]
Reference synapse detection program for processing serial electron microscopy data
3 stars, 3 forks
- 2015 CAJAL [URL]
A MATLAB API that provides a simple to use interface with Open Connectome Project servers and provides RAMON Objects, unit tests, configuration scripts, and utilities
6 stars, 5 forks
- 2012 – 2017 FlashGraph (Scalable Analytics) [URL]
General-purpose graph analysis framework that exposes vertex-centric programming interface for users to express varieties of graph algorithms
220 stars, 42 forks
- 2012 – 2017 FlashX (Scalable machine learning) [URL]
A matrix computation engine that provides a small set of generalized matrix operations on sparse matrices and dense matrices to express varieties of data mining and machine learning algorithms
220 stars, 42 forks
- 2011 – 2016 oopsi (Calcium Spike Sorting) [URL]
Model-based spike train inference from calcium imaging
20 stars, 9 forks
- 2011 – 2017 ndstore [URL]
Scalable database cluster for the spatial analysis and annotation of high-throughput brain imaging data
37 stars, 13 forks
Consultancy
- 2017 Consultant Greenspring Associates [URL]
- 2016 Consultant Scanadu
Advisory Board Appointments
- 2018 – Advisory Board Mind-X [URL]
A neurotechnology company combining brain-computer interfaces and artificial intelligence to make the world’s information available with the speed and ease of a single thought. Incubated at Camden Partners Nexus, completed an initial round of funding for an undisclosed amount. 15 employees.
- 2017 – Advisory Board PivotalPath [URL]
PivotalPath is a leading hedge fund research and intelligence organization built by a team of experienced alternative investment professionals and fintech developers. Raised undisclosed amount of funding, 11 employees.
Startups
- 2017 Chief Intelligence Officer sensie [URL]
Sensie is a startup devoted to unblocking intelligence to optimize our collective wellness.
21 employees.
- 2017 Co-Founder gigantum [URL]
The future of data science is open, decentralized and user friendly. That is why we created a platform that enables anybody to create and share totally reproducible computational work with the world. Completed initial round of seed funding for undisclosed amount from <a href="https://www.digital-science.com/">Digital Science</a>, which also funds figshare, readcube, altmetric, overleaf, and more. Gigantum was acquired by nVidia in early 2022.
15 employees.
- 2016 Co-Founder d8alab [URL]
Our services include evaluating model performance, building prototype R/Shiny web applications and basic data cleaning., Provides data science consulting for a variety of companies, specifically biomedical data science
4 employees.
- 2016 Co-Founder global domain partners
Global Domain Partners is a quantitative hedge fund that was acquired by Mosaic Investment Partners in 2012
6 employees.